Shakir Mohamed | 1345 words (~14mins). Will link to video and slides once it's ready.
for TEDxLSTM: Unknown Destinations
Dearest,
Dearest, note how these two are alike:
This harpsichord pavane by Purcell
And the racer’s twelve-speed bike.
The machinery of grace is always simple.
These are the opening lines from a poem entitled Machines by Michael Donaghy. For us here today, I hope they will foment questions of what it is to design, build and use machines-with-grace. The machines I’m thinking of are not the mechanical turks and automata of old. Instead, they are a new type of machine that is reactive, that assesses its own decisions, and that grows with experience. The machines i’m thinking of are machines with intelligence, machines that learn.
Designing machines that learn is the realm of the field of artificial intelligence and of machine learning. Like a knot, this field ties together strands from many different subjects: from statistics and logic and psychology and computation.
When we bring these different strands together we open up the possibility of designing these new types of machines: that can recognise objects and how they can be used, provide explanations of events and observations, reason about cause and effect, plan complex sequences of actions, and assess confidence in its decisions. These are elements of our human intelligence. And we could call a machine with these abilities, an artificial intelligence. Machine Learning gives us the tools with which to develop AI.
Although we don’t have any systems that can do all these tasks together quite yet, with what we already know, we can do much . As a machine learning scientist and engineer, I have been able to rely on the tools of our field to work on problems in many different areas: from systems that I hope could one day help us prevent organ failure in hospitals; all the way to those foundational questions of what might really be needed for machines to make sense of a dynamic world. In our field, we thrive on a sense of progress, our eyes fixed firmly to the future with optimism and excitement. Moving and forwards. Always.
Grace emerges in this movement. Grace lives in how we balance the role of new technologies against their impact on people and societies. Grace is in our memory of the change brought by past technologies, and in our consideration of safe change in the future.
Some of you here may have heard of the un-graceful, and at times simply disgraceful, uses of AI. Like examples of racially-biased face recognition that cannot recognise black faces, recruitment tools that discriminate against women, support tools for parole reviews that reproduce bias and injustice. Or examples of compromised security using DeepFakes that clone voices and faces from audio and video, or those systems whose accuracy is reduced by a simple adversary. There are many reasons for these lapses in grace. And perhaps part of the reason lies in the current state of global AI.
Global AI is far from global. Instead, it is localised and contained in specific individuals, organisations, and countries. And perhaps we can say this of global Science as well. We can better understand this by looking at the number of researchers in different countries as one rough proxy. Here in the United Kingdom, or in similar countries like Germany or the United States we find around 4000 researchers per 1 million people. In South Africa, where I am proudly from, this number is 400 per million. And in Ghana, the number is closer to 40 per million. The contributions to global knowledge are at present far from uniform. From this vantage, attaining grace remains in the distant horizon.
In machine learning, and similar areas where data is so tied to success, the generation, ownership and management of data is central. There are many important problems where pooling and using as much data as we have is needed for progress. But too often are there disgraces yet again: breaches of data privacy, or data being used for targeted misinformation, are just two that come to mind. And there are many grey areas, situations that support economic development on one-hand, while simultaneously invoking a tradition of extraction on the other. For example, it is not uncommon to now find large organisations in developing countries committed solely to the cheap annotation of data used for highly-profitable uses elsewhere.
If we put the global imbalance in the distribution of researchers alongside this view of an extractive role of data, you and I might recognise the creeping concern over a new type of technological colonialism: where resources and talent and money are taken and little left behind. You and I are not oblivious to the possibilities of imperialism based on data and its ownership. We can recognise a forming cyber-colonialism that expands as censorship increases and online freedoms are curtailed. As the critical scholar, we can do much more to add to this description of the global state of artificial intelligence and of our sciences.
Yet, countermeasures to technological colonialism are at hand. And together, we can enact them, by doing the work of Transformation. By Transformation I mean enacting the systemic and social changes that strengthen diversity, respect, responsibility and freedom - the essentials of grace.
While these might seem to be the grandest of ideals, transformation’s enabling principle is simple: Transformation lives in the mundane. Our work of Transformation lives in the everyday, in the small encounters, in our simple actions and reactions. When Transformation lives in the mundane it is magnified in the larger technological, social and institutional spaces we occupy.
This is then the call for a new Critical Practice of Machine Learning. A change to our practice that encourages our forward motion and optimism, while balancing our responsibilities to transformation and our communities. Forwards and balance—just like riding a bike.
And the beginnings of this work are already in place. We look out for solutionist thinking, knowing that the best solution for some problems might not need complex technologies. We admit that we cannot address complex social questions through mathematical formalisation alone. And we know that technologies beneficial in one setting and place, can be harmful in others. These are some of the principles that we put into the Dagstuhl Declaration on AI for Social Good.
There is one other solution from my experience that I would like to share. At its core, this new critical practice calls on us to develop open and genuine intercultural dialogues within our sciences. Dialogues that lead to co-design and inclusion in the mission of developing intelligent machines with grace.
One clear approach available to us is in our support of grassroots organisations and of their role in creating change, elevating intercultural dialogue, and in doing the work of true transformation. I have the honour to lead such an organisation, called the Deep Learning Indaba, whose mission is to strengthen machine learning across our African continent. Over the last three years, we have been able to build new communities, create leadership, and recognise excellence in the development and use of artificial intelligence across Africa. And I am proud to see other groups follow in the same vein, in Eastern Europe, in South-east Asia, and South America, all taking responsibility for their communities and building grassroots movements to support AI, dialogue and transformation.
I used the opening poem and its vision of harpsichords and bicycles and grace for two reasons. Firstly, to celebrate our progress in machine learning, but one that must now be balanced using a new critical practice. And secondly, for us all to think of transformation in the mundane, and the critical role grassroots organisations.
If we are successful in making global AI truly global, and I believe we can be, we set ourselves on the path to realising that intelligent machinery of grace. But so much more importantly, we will get there joyfully and together: at each step, moving and transforming our world with an ever-renewed solidarity and in friendship and with grace.
As our poem ends:
So much is chance, So much agility, desire, and feverish care,
As bicyclists and harpsichordists prove
Who only by moving can balance,
Only by balancing move.
Thank you.
END.
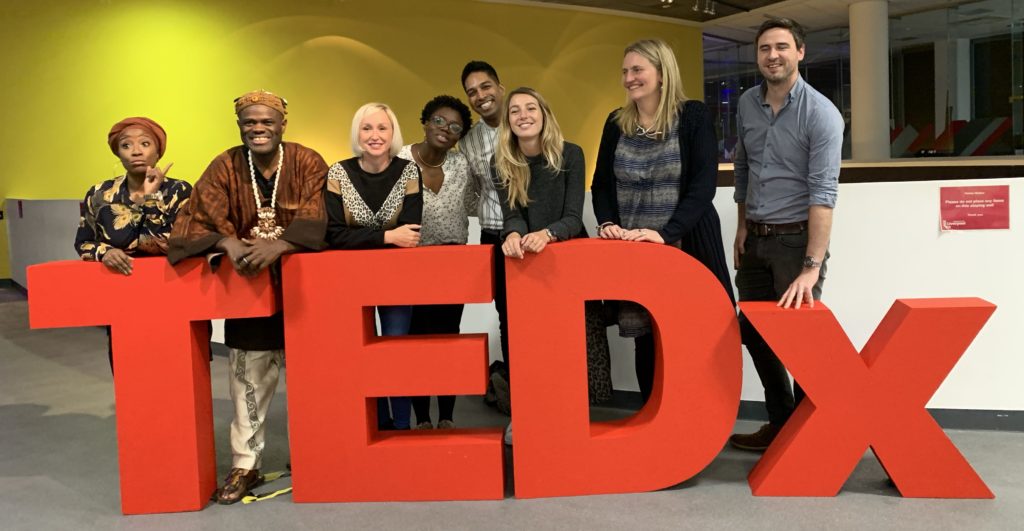