Keynote at the AI for Science Summit hosted by Accelerate Programme for Scientific Discovery. Video TBC.
Hello and thank you for having me today. I love coming back to Cambridge so it’s a real treat for me to be here today. Just looking at some of the titles of work that is being showcased at the summit today, I think we can say that many, if not most, scientific fields are asking what the precise role of new advances in machine learning and AI will be in advancing science and making research breakthroughs, if any. I can’t answer that question, but what I can do is demarcate a few themes and directions that underpins this questions, relying on my own experience and study. So that’s what i’m here to do today, to share my thoughts on this moment where generative AI might morph into a generative science, and to learn from your views on this.
Now before I dig in, let me say a little about myself. I am a graduate of this wonderful university. And today I am a research scientist at Google DeepMind, where I am a Director for Science, Technology and Society. So that means I work on areas where these topics intersect, and where machine learning and AI can be directed towards social purpose. Outside of that role , I am a founder and trustee of an educational charity called the Deep Learning Indaba, whose work is to strengthen African Machine Learning and AI, and which is doing the necessary work of growing who gets to be involved in AI developments and where. And then you’ll find me giving time to many other organisations where I can. I, of course, love speaking, and love exchanging ideas, so my thanks to all the organisers, especially Katie and Jess for the honour of this platform today.
Our new AI advances have many names, and we find ourselves using terms like generative AI, large language models or LLMs, foundation models, general purpose AI, AGI, and a swathe of other terms, to describe the types of systems that are have been put so forcefully into the public imagination, many times filling us with awe, and at others times with deep concern. Generative AI is a membership based concept, so it is defined by the set of approaches and applications that get put under that name. But if I am to offer some sort of a definition, generative AI centres on the role of forming and using probability distributions that are estimated from data. You can do this with varying levels of sophistication, but it is this probabilistic nature that is their success, and the reason why they are interesting for advancing science.
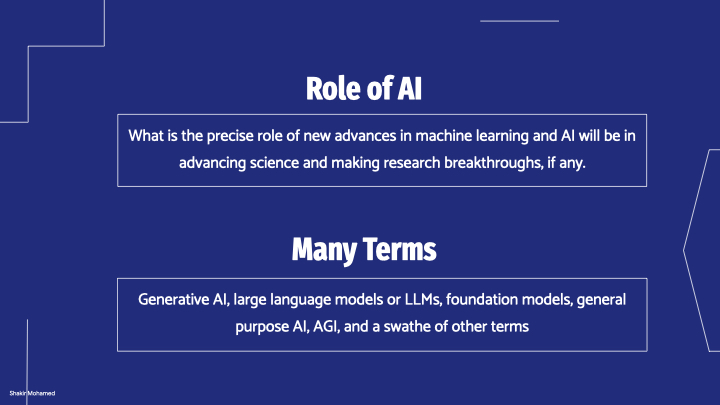
I want to use my time today to unpack a few different themes. We are starting with what is a seemingly anodyne question: whether and where is AI applicable in my scientific field. This question I think quickly unravels, leading to questions about the vigour and pace of scientific discoveries, about the role of theory building and data, and of measurements and rigour in assessing scientific progress. AI is itself, partly, also a scientific field, so those same questions apply to it. Beyond this, there is the direct question of how language models and multimodal models might change, enhance, or ruin how we engage with the rich body of literature and research available and the synthesis of scientific knowledge, which is the first step in the methods of producing new knowledge. And then there are specific technical questions like how scientific simulation that underpins many areas of science is changing and what that means for basic research and the way science informs and shapes our commercial, environmental or societal priorities.
So together there are 4 parts and 4 discussion topics I want to open up today: one, on Progress and Pace in Science, and topics on measurement and evaluation; two, on LLMs and Science Assistants; three on scientific simulation, where i’ll describe advances in weather forecasting; and finally 4; on scientific responsibility. You can treat them all as separate topics, but I hope by the end they will all come together into a whole and we can pick apart any arguments in the panel discussion.
Part 1: Progress and Pace in Science
This part is meant to put out some strategic considerations, and to think about what is motivating us to think of generative AI applications in the sciences. To do that I want to start the two quick topics on things coming to an end.
The End of Theory
Every few years a provocation flows across our sciences declaring that we have reached the end of theory. Or as this headline asked, are we now in a post-theory science. Since we are able to collect more and more data, it became natural to ask if we could ‘let the data speak for itself’, obviating the need for theories underlying the processes the data was reflecting. By collecting ever-larger amounts of unstructured data and logs, we could skip the hypotheses, since data and its metrics and summaries could tell us about reality directly.
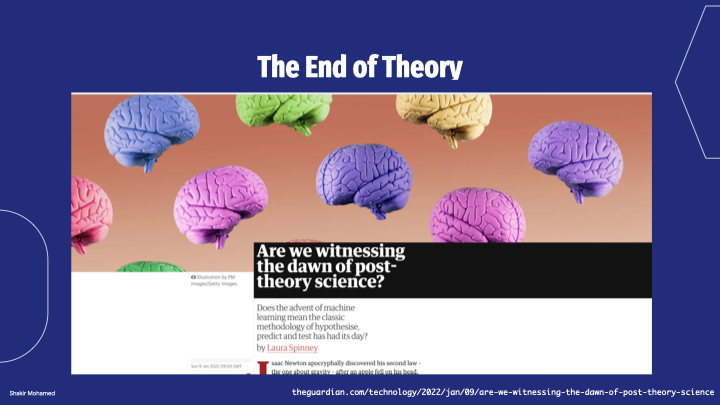
Now, data might be able to make this possible - scaling laws for large language models and ever larger generative models and their impressive outputs might suggest this. I think this is one part of the intrigue of generative AI for science. We know that many scientific fields are generating immense volumes of data, data that can be highly complex, where apriori hypotheses are hard to come by, and where that data could be essential to new types of resilience and long-term decision-making. And this exists alongside the pragmatics of throwing away data in high-throughput scientific fields because it's difficult to store and because of a lack of useful models and tools to extract insights. So maybe generative AI applications are part of this drive for a post-theory mode of science.
But even the most removed of researchers today are aware of dataset bias, sampling bias, issues of calibration, the problems of unfairness that emerge when we use data without questioning what and how it was collected in the first place, and how these basic problems can remove our hopes for positive impact, and instead, cause harm; in addition to forgetting data processes being methodologically weak. Given how serious a set of concerns these are, it’s safe to say that the end of theory and the imaginary of letting the ‘data speak for itself’ won’t stand up to the light of this scrutiny, at least not for now.
Keep this topic in your working memory, since i’m bringing it up to provoke some thinking from you about the kinds of questions we ask using our data, and importantly how we go about looking at the answers we get, and how we measure our outcomes and achievements.
Now a second ending.
The End of Invention
While post-theory is being debated, the last few years have also raised another question about the end of invention. Or more specifically, the sense that scientific discovery and innovation has been slowing down and significantly so. I’m sure as members of the university, this is a topic of regular discussion. One story that can be told using a set of different measures is that science is slowing down: knowledge is today immensely complex requiring more time in any single field to build a mastery of, PhDs take longer to complete, the time to publish first books takes longer, the time to receive first grants takes longer, and while the number of people working in scientific fields is increasing some measures say that research productivity is plummeting.
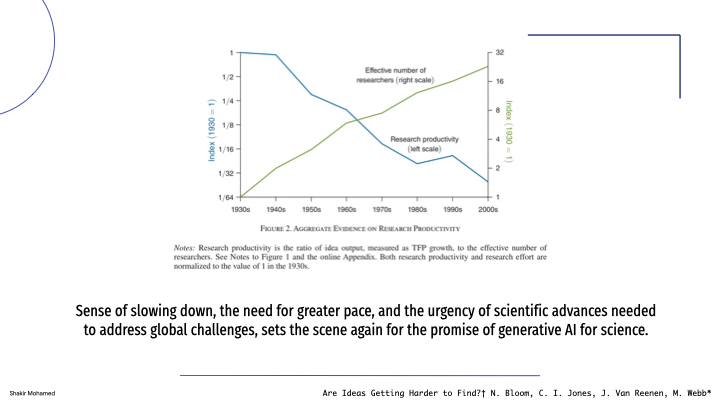
I wouldn’t fully lean into this narrative, but this sense of slowing down, the need for greater pace amid growing complexity, and the urgency of scientific advances needed to address global challenges, sets the scene again for the promise of generative AI for science. This generative science, it is hoped, can add vigour into the sciences, especially in cross-disciplinary ways and provide broad-based benefit.
The reason I bring up this story is to again provoke your thinking about why and where we think generative AI will have value in the sciences. And again, if we do want to increase pace, part of that drive will be deeply connected to how we measure and report progress.
So let me say something generally about measurement.
Measurements and Theory
Science and measurement are intertwined with each other. In any discipline, scientific progress is strictly limited by the capacity to measure relevant concepts. In creating these measures and metrics, we are creating new theories. I’ll also say the obvious as well: that things we want to measure, and the measurements we make, are not always, or even usually, the same thing. Because of this, we have to think about our measures, and because we can regularly get our measurements wrong, there is at times a tenuous link between measurements and impact.
But I do think, across all computational fields of science, we question our data and performance measures all the time (hopefully). And this process of asking what is and isn’t in our assessments, conveniently and unsurprisingly, is itself also a process of creating and testing theories. A theory here isn’t just a set of equations describing a phenomenon, but can also be many other things, like a description or a process, a set of assumptions, and tests and criteria. So what is clear then, is that theory is still very much active and that, actually, we are all theory builders, and that we are doing it every day. The science of machine learning especially is still one that is all about theory generation, falsification, and regeneration.
Measurement theory is the subject and set of tools that encourages us all to think about the meaning of our metrics and measures. It encourages critical assessment of the assumptions behind our analyses. And in so doing, it encourages responsible real-world data analysis. So a crucial part of a safe and responsible AI effort for us, starts with our metrics. But that, I think, is part of the exciting work of machine learning now and in the future.
Measurement theory raises questions about the generalisation of a metric across conditions and scenarios. What coverage does a metric have in the space of problems that we study. What aspects of convergence or divergence arise when it is used. What different types of measurements and data can be combined to reveal the impact we want to have.
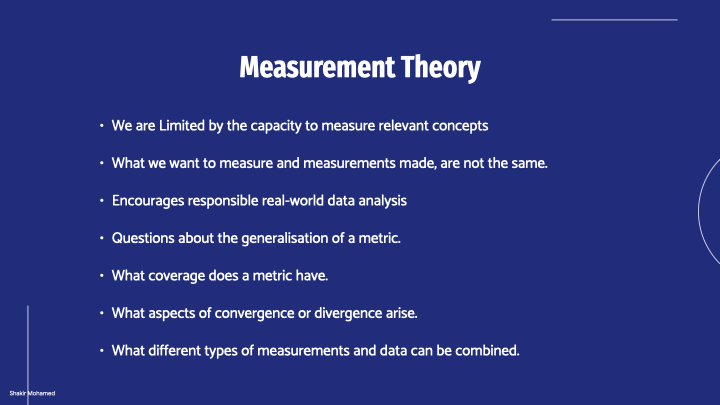
So let’s establish two conceptual framings that will prove useful . The first conceptual frame is around the type of evaluation. In machine learning, you could say we have two types of evaluations. There are the design-time evaluations, which are the measures and assessments we use to train our models, choose hyperparameters, do model selection, and design better models. And then there are the run-time evaluations, which are assessments outside of training, about how a model could be deployed and will perform in different scenarios and with real users and interactions. These two are of course connected and inform each other.
The second conceptual frame will be the scope of evaluations. Evaluations in our discussion need to go beyond technical measures, and I want to expand what we consider to be the scope of our evaluations. The key dimension along which this scope will increase is in the role of people. People includes us as researchers, people in other fields, sectors and industries, but also publics and people across the world.
This leads me to one conclusion I’ want to leave with you, which is in the use of what are sometimes called mixed-methods of measurement: the use of deeply technical, automatic and quantitative assessments combined with qualitative and people-focussed methods. Their combination can reproducibly lead to clearer views of how a system works and the gaps between desired and actual impact.
In the discussion, I’d love to hear what tools and approaches for designing measurements you consider and use in your research. The wondrous thing of AI for Science, is that we can bring very divergent approaches to evaluations together: relying on centuries of experience in scientific domains, alongside new large-scale evaluations from data science and AI.
To summarise the point so far, let me invoke the words of Wangari Maathai, the nobel laureate and one of our world's great environmentalists. Her words capture the theme and interconnectedness of topics I want to leave you with today. As She wrote: “The task for us all in healing the Earth’s wounds is to find a balance between the vertical and horizontal views; the big picture and the small; between knowledge-based on measurement and data, and knowledge that draws on older forms of wisdom and experience.”
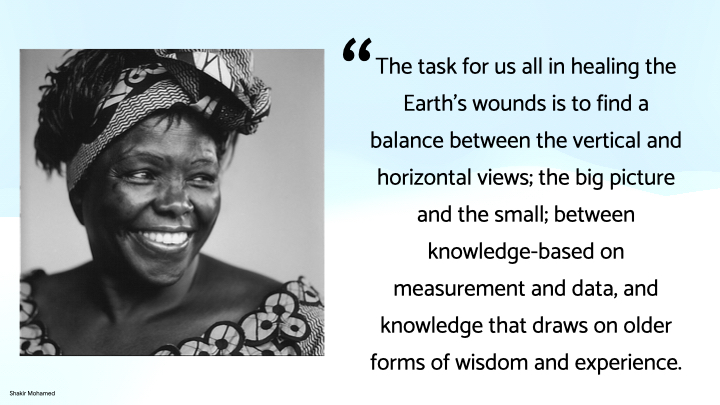
So this part started off with some big picture questions all about pace, progress and measurement. I’m sure we can spend an hour talking just about this, but for now reset your attention, and let’s start afresh on a new topic. So onto:
Part 2: Science Assistants and Knowledge Production
I have a question - and I’d love to hear some answers from a few of you. Do you use generative language models in your research? What for?
Science Assistants
Following from the topic on the end of invention, if we want to tame knowledge complexity, then one solution seems to be to create tools that help us better and more effectively navigate the immense set of knowledge available to us, by summarising data, bringing disparate and previously unconnected sources together, and tying threads of evidence together across studies. And this seems to be something that language models right now might actually be able to do. And of course there are now many attempts to create these types of science assistants:
- There is galactica, an LLM that aimed to be a general science assistant, to help us all get a better grasp on all papers we wish we could read.
- There is med-palm that is a form of medical assistant.
- The recent Gemini release showed an application for scientific summarisation and data fusion.
- There are code assistants like github co-pilot that help us all write code faster.
And so many others. All have varying degrees of success and none yet been proven thus far.
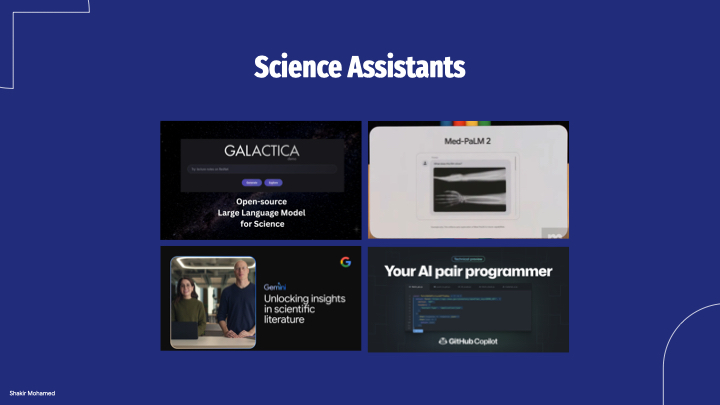
There is a lot here for us to think through in what is useful and not, so i want to bring up a few such questions to interrogate in the panel section.
- Many evaluation approaches right now take these tasks of answering questions, summarising information, and showing mastery of a field, by measuring performance on standardised tests. What other evaluations beyond this are going to be needed?
- What are the sustainable, respectful and cooperative ways of bringing experts and scientific communities into the design and use of these models, and how do we make these tools more people-centred?
- Factuality and grounding remain unaddressed in these models. So they are a critical priority for everyone developing those methods. But as long as these models are only generating statistically plausible sentences without reasoning or groundedness, this will only create more work to assess correctness and usability (and perhaps slowing us down further). What is plausible for us to do with these tools today, and how to ensure accountability?
- Research and science I believe is still a creative process. That process involves knowing what has been done before and honouring that work. It involves gaining an understanding of where a frontier of knowledge is, involves reasoning about the tools available today and how they can be extended to expand a scientific frontier, and it involves us understanding the institutional and social context we are in to decide on which of the many different directions we should put our efforts into. LLMs don’t do reasoning right now, they don’t have this broad contextual framing (right now at least), and the work of science remains fundamentally human in this sense. In what ways do we expect this to change or should change?
Likelihoods
Although I want to keep my focus more high-level, all these questions are again ones of creating better evaluations. So let me take this opportunity to epmpahsise the importance of foundational machine learning research in this regrard.
As a short technical deep dive, i want to make the case for you to think about the role of likelihood evaluations in your research domains. Likelihoods are the basic tool of statistical fields and, for many of us here, the basic tool of measurement we use in our work is some form of likelihood (either as an approximation, a lower bound, or a perplexity). Likelihoods are our superpowers as statisticians.
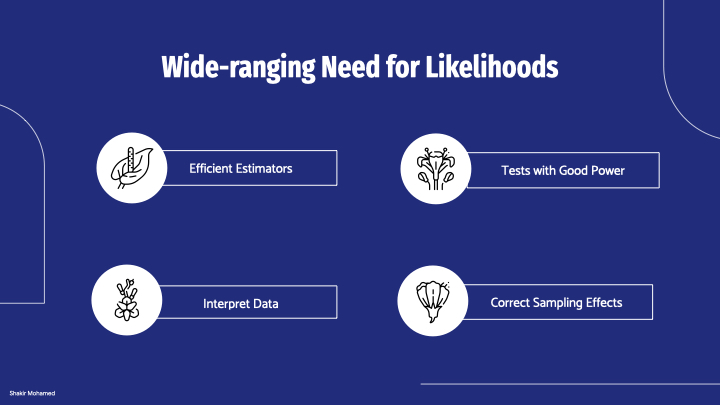
If we can compute a likelihood—so in some way know the probability of our data—we can use it to build efficient estimators, develop tests with good power, and understand the contributions individual data points make to our predictions. We can use likelihood functions to pool information from different sources or data, and can take on creative ways of handling data that is incompletely observed, distorted or has sampling biases. Likelihoods are an amazing tool for both learning and evaluation, for both design-time and run-time. But I want to emphasise their role in the evaluation of deep generative models.
Generative models learn distributions of observed data. Some classes of probabilistic and generative systems make the computation of likelihoods possible to evaluate or approximate directly, like graphical models or factor graphs. Other approaches, like generative adversarial networks or diffusion models, do not make computation of exact likelihoods easy or possible. Regardless, assuming you could estimate likelihoods in a probabilistic model, what are the things we might want to do?
Generative models learn complicated, multimodal distributions, and we know they will be misspecified, so we’d like to use the likelihood to understand how our models are misspecified. We will want to know:
- Which modes have been dropped in a learnt model.
- Do we have an imbalance in the modes we have learnt.
- And in any specific mode or class, how well did we capture the diversity of data within it.
To answer these questions, we’d need some type of likelihood estimation method that does the following:
- Assigns probabilities to every data point individually.
- Allows us to include label information, if we have it.
- Is computationally tractable.
- Can be applied to a large array or models. In the most general form, we have a method that only needs samples from a generative model.
With these desiderata in mind, one very flexible tool you can look at is the Empirical Likelihood. The empirical likelihood is not often studied in machine learning, but one I hope many of you will pick up on. It is a classical non-parametric method of statistical inference, and it will be intuitive. The classical empirical likelihood is a method for inference on the mean - so that is a way of doing a statistical test of whether we estimated a mean correctly. I’ll leave a pointer to a paper that describes in detail the evaluation of generative models that develops a measure of the generalised empirical likelihood or GEL that will meet all the evaluation desiderata we had, and builds on existing methods from the field of representation learning. We need many different methods for evaluation, so I can only encourage you to give some of your time to research in this area.
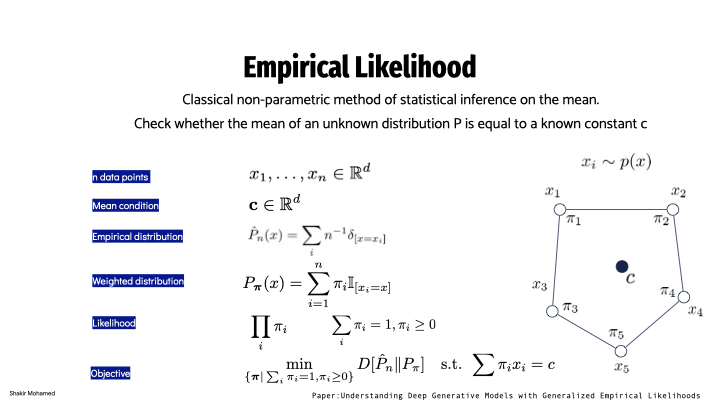
So this part was about language models as assistants in taming knowledge complexity, and on developing foundational tools of assessment. Let’s reset again and this next part will be about generative AI directly in fields of science. So:
Part 3: Scientific Simulation
One element of many sciences, especially the physical sciences, is the role of describing the world using a set of equations and then simulating future worlds by numerically solving these equations. Scientific simulation of this form is everywhere and a bedrock of science and engineering. Just look at the the topics we heard about today, understanding oceans, breaking waves, the cell, biodiversity, high-energy physics, and more: simulation is everywhere.
Machine learning becomes interesting here because it changes how we look at the problem of scientific simulation, by transforming the problem of high-dimensional numerical solving, into a problem of learning from simulation and observational data. And when the problem becomes one of learning, we can open up new research frontiers and opportunities.
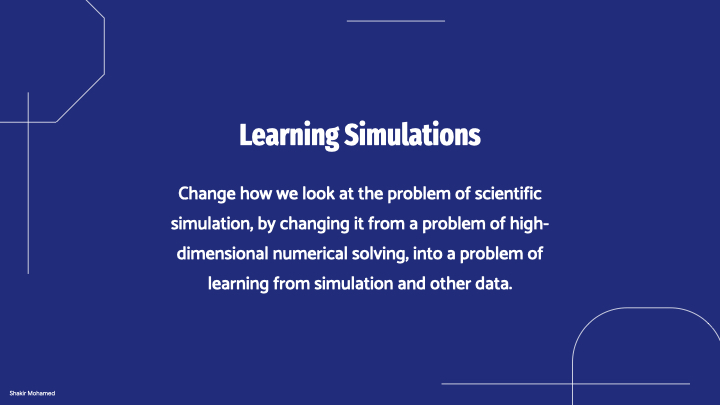
Earth System Modelling
I’m going to use the problem of modelling the Earth system as a case study for our discussion. The Earth System is a representation of all the processes on our planet. That includes the clouds and rain, the wind and sun, the oceans and land. Typically we can represent the earth system using the physical equations that describe the state of the world. Earth system models are everywhere: they are of course the tools that continue to deepen our understanding of this changing planet we inhabit; and they are central to our ambitions to live sustainably together. This is a really a fun area to work in, so please join in.
The modelling of weather and climate processes is a marvel of science. These models are general in their uses and directly inform decision-making across sectors. Decisions are taken by people, and the impacts and purposes of weather and climate modelling are made clear when we look at what those decisions are. And one common framework is to decompose these decisions along the prediction horizon that is used.
- There are many critical decisions that support emergency services planning, flood response, outdoor event planning, or other activities where the protection of life and property is urgent. These typically depend on regional predictions that are 1-2 hours ahead, which is referred to as nowcasting.
- Yet, other decisions need more advanced notice. Load balancing on power grids where power generation is spatially and temporally optimised, management of renewable energy sources, and national weather operations work on time scales from a day up to 10 days ahead. This requires medium-range forecasting, that makes predictions of all atmospheric phenomena and typically across the entire earth.
I’m going to focus on this medium-range scale, but there are other important timescales, namely, Sub-seasonal to seasonal (S2S), Decadal climate forecasting, and, multidecadal projections, or more simply climate projections.
Medium-range forecasting
Medium range weather forecasts are truly incredible feats of numerical and statistical forecasting. Weather predictions are ones we all rely on every day, directly or indirectly, and with applications wherever we have commercial, industrial or social needs. Right now is an incredibly exciting time, since Machine learning is making significant inroads into this area.
For medium range forecasting, the prediction problem involves making predictions in 6 hour intervals from 6 hrs to 10 days ahead, at around 25 km spatial resolution at the equator. In the data we used, each “pixel” in this grid on the earth contains 5 surface variables, along with 6 atmospheric variables each at 37 vertical pressure levels, for a total of 227 variables per grid point. So that means 235k variables for each time point we want to make a prediction for.
One magic ingredient here is the commitment of international meteorological organisations and their member states to making numerical simulations available for us to use as training data, specifically a type of data known as reanalysis data that covers the last 40 years. The aim here is to build the best general-use base weather model we can. This base model can later be built upon by other users, like environment agencies or commercial operators, for their specific problems. And these problems are many: renewable energy, logistics, aviation, floods, safety planning and warnings, and so many more.
To show how this works, it is common to produce a scorecard, which is what you are seeing on the screen, that visually summarises model performance across different variables, and using different metrics; blue squares when the model is better than the operational system, and red when it’s not. Importantly, they also show performance across different subsets of the data, like performance for the southern hemisphere vs the northern hemisphere, or other important subsets of the data. This scorecard shows that machine learning approaches can outperform operational weather systems. And so with this scorecard, we have affirmatively answered that long-standing question of whether data-driven machine learning approaches for weather forecasting can be competitive with world-leading operational forecasting systems.
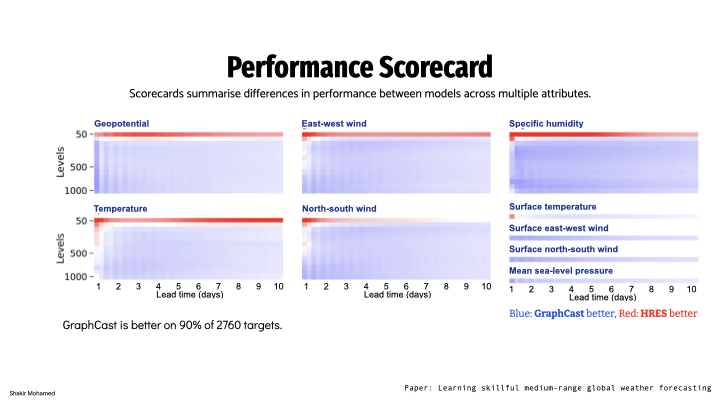
Using graph neural networks, we are able to show state-of-the-art performance that significantly outperforms the most accurate operational deterministic global medium-range forecasting system on 90% of the 1380 verification targets we assessed. This model also outperforms other machine learning-based approaches on 99% of the verification targets that are reported. Our model can generate a forecast in 60 seconds on a single deep learning chip, which we estimate is 1-2 orders of magnitude faster than traditional numerical weather prediction methods. To show that these models are effective we assess them on their skill in forecasting severe events like cyclones, atmospheric rivers, extreme heat and cold, and others.
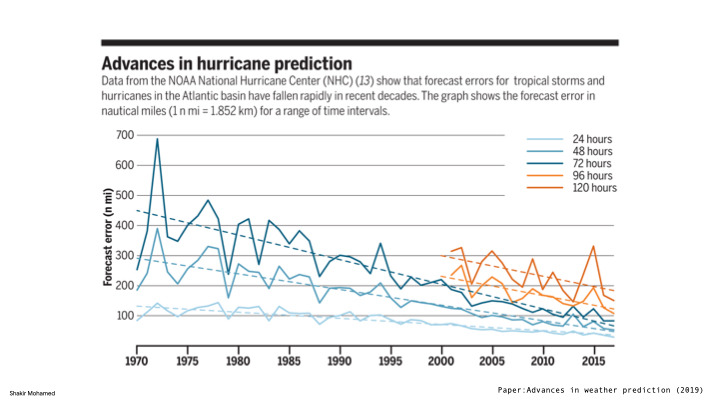
I want to zoom in on hurricanes specifically for a bit. As context, the 3 day ahead predictions of hurricane tracks we have available today, are more accurate than the 1 day ahead forecasts we had 40 years ago. So this technical field has increased both accuracy and the prediction horizon of its forecasts, while enabling all the safety and social benefits that forewarning brings.
Some of the generative science possibilities came into view a few weeks ago with the formation of a storm system that was later named Hurricane Lee. The ECMWF, widely recognised as the world's leading centre for medium-range forecasting has now made available several of a new generation of machine learning-based approaches for 10 day ahead weather forecasts. The availability of these forecast models allowed people who work on hurricanes and extremes to monitor and record the performance of these new machine learning predictions, and specifically predictions for wind and sea level pressure, which are key variables for analysing these storm systems.
When we assess over the collected set of cyclone tracks collected in a common hurricanes tracks dataset, this approach gains around 9hours in accuracy over the operational baseline.
Looking at a second severe event prediction: Atmospheric rivers are a weather phenomenon that transports large amounts of water vapor away from the tropics, and can cause heavy rain. So they are like ‘Rivers in the sky’, and one way to assess these is to compute a statistic known as the Integrated Vapour Transport (IVT). This approach gains around 1 day of accuracy over the operational system when forecasting IVT.
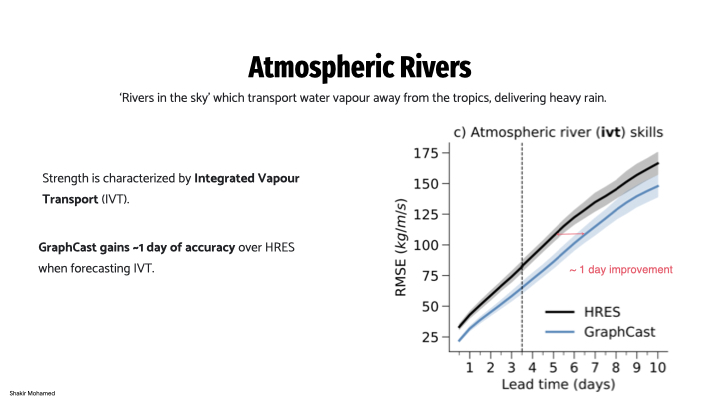
And finally, we can look at performance in predicting the top 2% of surface temperatures. The approach we show has better performance at long-lead times beyond 12 hours compared to the operational baseline.
Now, I don’t want you to leave with the impression that we now have a replacement for widely-used numerical weather prediction methods, or the role of physical knowledge and simulation. Rather, what’s clear from the results of so many different contributors and machine learning groups across the world working in this area, is that rapid advances are being made in the use of machine learning for weather. We are seeing this in improvements compared to operational forecasts and across forecast horizons, and we hope this is part of opening up new ways of supporting the vital work of weather-dependent decision-making that is so important to the flourishing of our societies.
As a quick plug, if you might have work in this general area, do consider submitting it to the ongoing JMLR special collection on Machine Learning addressing problems of Climate Change.
Part 4: Responsibilities of the Pioneer
Stay with me and let’s reset one last time, so we can talk for short while about the changing landscape of responsibilities for the scientist.
While so many technical advances continue to be made and positive outcomes are expected, they often lead to the opposite. To bring an example, improved forecasts of El Nino are now available, and with that a new tool to assess shipping conditions and potential changes in ocean fish populations. This was a potentially socially-beneficial use case for the fishing communities of Peru in 1997. But fishing companies, incentivised by the prospect of a weak season based on such forecasts, chose instead to accelerate layoffs of their workers. So instead of benefit, here is a case where improved forecasts have led to demonstrable harms and the opposite of their assumed benefit.
If I give you a quick second example, today, the average forecast accuracy for high-income countries is more than 25% higher than the average accuracy in low-income countries. Making the problem of forecast equity a distinct challenge for us.
We spoke already about concerns of scientific misinformation from the use of language models with no grounding in actual scientific basis or the ways that uncertainty is navigated in science. There have been many examples in the media in this area that might be useful for you to bring up and add to our discussion.
What all the examples reminds us, is that we are not merely passive observers of the new horizons in generative AI for science, instead we are those people defining what the frontier and those new horizons will turn out to be. And it is for this reason why I’m identifying us as pioneers, and as pioneering. And if we are pioneers, I think that gives us some foresight, some power, and ultimately, some responsibilities.
We can’t not simply assume that new models of AI and weather, or any other area of Ai for science, will have a positive impact and be used for outcomes that lead to prosperity. In an famous essay entitled the responsibility of intellectuals, Noam Chomsky wrote something very relevant for us, that “it will be quite unfortunate, and highly dangerous, if they [new advances] are not accepted and judged on their merits and according to their actual, not pretended, accomplishments.” Creating the culture for this form of accountability becomes one of our responsibilities as a field of AI for science.
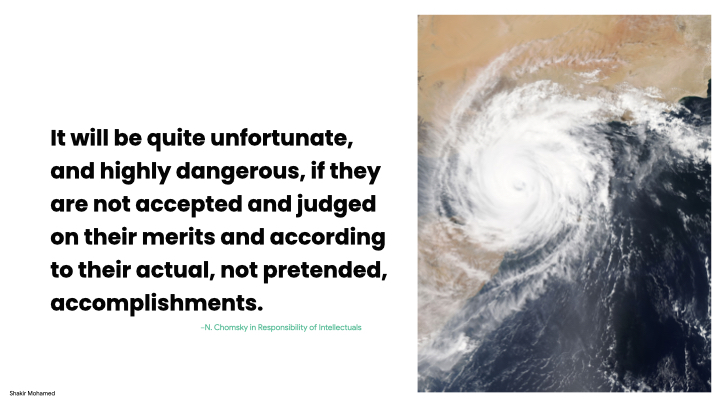
Wrap up
I bombarded you today with a lot of seemingly disconnected topics, but they were all related to the focus of the summit today. I wanted to be broad so that we have a lot of directions to take in the panel discussion and over dinner tonight.
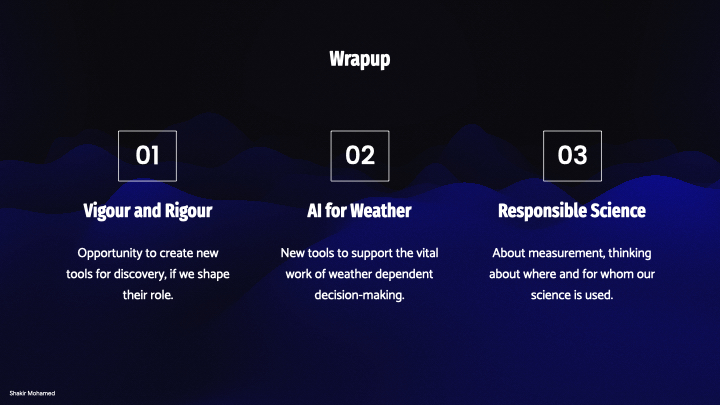
If i achieved my aims then you got to this point with three key outcomes
- Vigour and Rigour. Generative AI for Science presents opportunities for new ways of doing theory and renewed vigour across our sciences. There is an amazing opportunity to create new tools for discovery from large amounts of data, faster simulation, or taming the deluge of papers, and tools to support our work and productivity; if we shape their role in support of people and society.
- AI for Weather. Earth systems is a wonderfully exciting field to work in. There is a space for many nature and science papers here, and to support the vital work of weather dependent decision-making that is used every day.
- Responsible Science. The responsibilities of scientists are broad, and more than producing new knowledge. For us we need to maintain a focus on measurement, thinking about where and for whom our science is used, and create new cultures of curious and responsible science.
I thank you all for having me today, and for allowing me to work through my own thinking with you in this way. My deep thanks to you all.
As a short postscript, here are some resources to followup on:
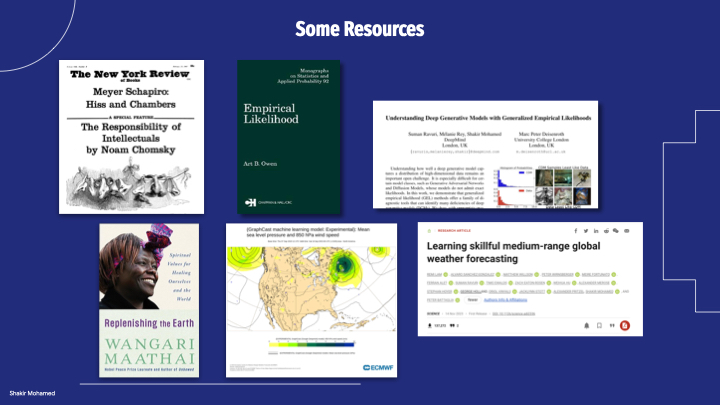
- Noam Chomsky’s New York Review of Books essay on the Responsibility of Intellectuals.
- The wonderfully written and comprehensive book on Empirical Likelihood by Art Owen.
- And the lastest machine learning based forecasts deployed by the ECMWF, which includes the graphcast model I referred to, at charts.ecmwf.int
And some papers I have been lucky to be involved in with many amazing collaborators:
- A paper on generative model evaluations, Understanding Deep Generative Models with Generalized Empirical Likelihoods.
- And Work on 10 day weather predictions, Learning skillful medium-range global weather forecasting.
Thank you all again.