I am incredibly humbled to have been able to give a short talk at the Queer in AI workshop at ICML2020. This is the text of the talk. Watch the video. Look through the slides.
What an experience we are all having in recording these videos! Of all the videos you could be watching, Thank you for watching this one, and being here - I’m honoured to be given the gift of your time. And of course, a huge thank you to the organisers of the ICML2020 Queer In AI workshop for this opportunity.
I’ve entitled this talk ‘Queering Machine Learning’, which is theme I want to explore with you today. To get us started I’d like to provoke your thinking with a question.
Why are we here at QAI? Or asked in another way: Why do we gather and organise ourselves in this way? Why do we organise?
Interpret this question in as broad or narrow a way as you like. And please re-form this question for any and all of the other communities you belong to---black in AI, WiML, the Deep Learning Indaba, Khipu---and any others you find filiation with. Since you are watching a recording, indulge me by pressing the pause button to take 2 minutes to write down any keywords or reactions you have to this question. Why do we organise?
Based on what you’ve just written down, I hope that what I will speak about for the next 15mins or so will have some resonance. And I hope we can use your responses and reactions as a theme for follow-up discussions throughout the week.
Allow me to introduce myself briefly. My name is Shakir Mohamed; I use the pronouns he/him/they. I am incredibly grateful that the journey of my life so far has led me somehow to the point where I can be engaged with you in this way. In fact, that is what Shakir means-it’s an Arabic word for a grateful person. I am a proud South African and was born during the late apartheid era, and also born into the religion and traditions of Islam. Much of my memory is of the city of Johannesburg, where I was born and bred and lived until I left to do a PhD in Machine Learning at the University of Cambridge. So these are some of the preconditions for much of my journey towards self-confidence, and ultimately self-love---involving all the usual suspects of crises of intellect and faith and body and sexuality and racialisation and culture and place. Today, I am happily quietly confidently queer. These earlier crises do still resurface, but in different ways, and still being unsure, each day I continue to explore my place and voice in this world. So all this is to draw you closer to me, and with that bond start a conversation about our responsibilities and roles as queer scientists and citizens.
Let me now pose a very different question to you. We are together at a virtual conference on machine learning. So, What is machine learning?
Again you can indulge me by pausing the video to jot down some of your own thoughts. And I hope you’ll use the chat to share some of your answers to this question with others.
I’ve asked this question many times, and it is a surprisingly difficult one to answer. I suppose this reflects just how multifaceted machine learning is as a field. One of my answers to this question is to describe machine learning as a process of creating pathways from principles to products. This is an attempt to recognise that our field is both a scientific field, committed to advancing the statistical and theoretical basis of learning from data; as well as an engineering field, working towards building systems that can be deployed in the real-world and that adapt to the needs of noisy, complicated, impactful problems.
Inspired by many of the conceptual hierarchies we use in computer science, hierarchies like the classical cognitive architecture, Marr’s levels of analysis, or Sun’s phenomenological levels, I sometimes describe machine learning’s pathways using my own four layer hierarchy.
- At the bottom are the principles upon which our field is built. Those questions of probability theory, mathematics, asymptotics, neuroscience and cognitive science, and many other subjects.
- Building on that we have informational questions. This is where questions of prediction, uncertainty, and learning with depth emerge.
- This then leads us to more complex questions of reasoning, whether long-term planning, world-simulation, or explanation.
- And at the top of this hierarchy are applications, in healthcare, environment, and many others where we believe machine learning can have utility, and that motivates so much of our work.

In my own work in variational inference I have begun right at the bottom as we were thinking about questions of approximate Bayesian inference and the differences between implicit and prescribed generative models. And in other cases, I have started right at the top, as we took on the challenge of developing systems to support the early prediction of organ damage in hospitals using electronic health records. Each of us will start at different places in this hierarchy, and at different levels, and then access different parts of it in different ways. This opportunity we have to switch methods and tools and perspectives is, for me, why machine learning is so exciting a field to work in.
What I hope you will see when we observe our work using this hierarchy, is that the questions we ask are for the most part epistemic questions. They are questions of knowledge that we think are important in our field and that drives what we work on and consider state of the art. This hierarchy reflects the epistemic values we use in our research - values like reproducibility, the role of depth and attention, importance of calibration and uncertainty, a focus on reinforcement learning in developing intelligent systems.
This hierarchy though is deficient in one significant sense. This hierarchy fails to recognise that our field is not shaped solely by epistemic values and questions. Like all other fields of science and technology, our work is also shaped by non-epistemic values, or more simply, contextual values. These are the social, cultural and political values and considerations that we all bring to our work in machine learning. This is where our queerness enters.
Queerness in science might best thought of as a verb: to queer. When we queer something, we use the human experience of desire and identity and relations to show that concepts and tools that we take for granted, might be the opposite of what they appear to be. We are able to reveal that our work and methods and thinking already has far more variety than what is visible or mainstream. This is a queering of machine learning, and a powerful tool of self-refelection; an approach to machine learning research that is more critical and responsible; a tool available not only to queer researchers, but to everyone.
I think we have enough evidence in history of what is possible when the queering of our world is taken seriously.
Remember the work of Edward Carpenter, the late Victorian intellectual and activist, who used his queerness to work against imperialism and reveal it for all its evils. You’ll recognise him as the inspiration for EM Forster’s book, Maurice. Especially in this time, where we are rising up globally in support of #BlackLivesMatter and against the ills of racism that is a direct consequence of slavery and empire, we find that queers and queerness, despite all the challenges they themselves faced, were allied to the cause of racial justice and equality from the earliest times. This is a demonstration of the important principle of solidarity and collective strength, and an inheritance that is ours to use.
That Black lesbian mother warrior poet Audre Lorde, in the opening lines of her poem A Litany for Survival, wrote:
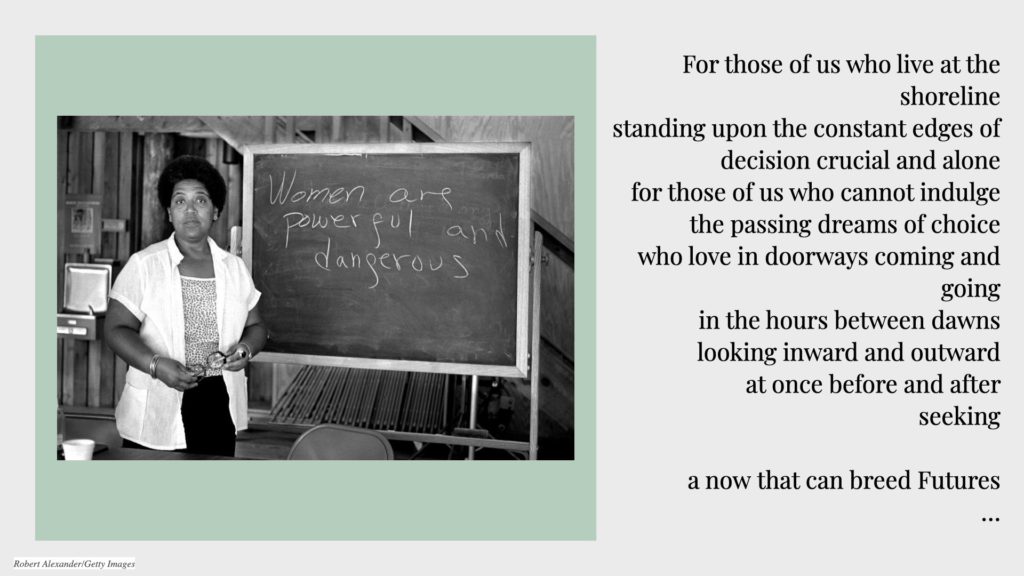
Her message is as relevant today as it was when she first wrote this poem. Her activism, courage, and writing changed the world, and reminds us that seeking other futures is what we, who live at the shoreline, can and must continue to do.
The queer activist Simon Nkoli proclaimed at the dawn of South Africa’s democracy:
“In South Africa, I am oppressed because I am a black man and I am oppressed because I am a gay man. So, when I fight for my freedom I must fight against both oppressions”.
Simon Nkoli, Joburg Pride 1990
Simon died of HIV/AIDS in 1998, and thinking of him reminds us that by queering the values and systems we exist in, we can change the shape of entire democracies. Here, I'm thinking of this influence on rights enshrined in the South African constitution. But I’m also thinking of the many incredible people, like Binyavanga Wainaina from Kenya, who sadly also died last year, Jason Jones from Trinidad who is reshaping the rights of millions across the Caribbean, the Tongzhi in China, and so many others queering for their rights.
I think we have also seen what happens in a world that denies queerness. One example that always comes to mind is Darwin’s work, The Descent of Man, and Selection in Relation to Sex. Because of the prevailing cultural attitude of the time---i.e. Victorian contextual values---this work failed to recognise the same-sex behaviour observable in the animal kingdom and the long established variety in human desires, so instead, was only able to conceive of a role for men in relation women, and became a manifesto for heteronormativity and gender hierarchy. This is a failure and the consequence of what happens when we fail to question the contextual values embedded within our sciences.
We can already see this same type of failure beginning to manifest in machine learning as well, with examples abound. There is the infamous case of the image classifier developed to categorise people as gay-or-not from their faces alone. What makes that scene worse, is that the researchers involved remained seemingly oblivious to the possibility that their work was highly suspect, and that they had fallen into the trap of essentialising gay people.
Other examples include: the natural language tools that classify queer vernacular as toxic in online forums, trans bodies that are systematically singled out by airport screening systems designed only for a gender-binary world, and in questions of algorithmic fairness that are currently developed while restricted to binaries of gender and race. And these are amongst many other emerging issues ranging from the search for gay genes in genomics data, the online tracking and doxxing with the intention to out, discredit or intimidate, all the way to discrimination in hiring and the provision of social and healthcare services. The queering of the technical landscape of machine learning is one important response to that question I posed to you at the very beginning.
There is another, perhaps more important, response to my opening question of why we gather and organise. For many of us, I suspect the answer to that question was instinctive: to belong. If we think of all our traditions and oral histories, of the great works of literature that record life and culture, and think of all our emotional reactions in times of joy or crisis, one thing stands out, and that is a constant search for belonging. In being together, there is a connectedness and a collective confidence that we build. And In being together, we make it possible to create a new language with which to name the coming technological challenges I alluded to earlier.

But belonging has never been easy, or free. Especially for queer communities, where so many know what it means not to belong. Not belonging in school or university is hard. And how much harder it is, to go home and still not belong. To not belong, is to be alone. And loneliness is a major issue facing queer people, manifesting in many ways, whether in violence, high incidences of mental-health cases, drug-abuse, or high rates of suicide.
The paradox is that loneliness and belonging, go together. That’s because genuine belonging, truly genuine belonging, always allows people to be alone--the better word to use here might be solitude. It is in creating this type of belonging where many communities fail. This is also a deep point about inclusion that is often overlooked in so much of the work on D&I-Diversity and Inclusion. When we truly and genuinely belong, no matter how divergent my opinion might be, how critical a viewpoint I might take, or in the face of the mistakes I might make, I will know that I still belong, and so do you.
This form of belonging is crucial. Because it is only with this type of safety that we can be more critical of the work we do, and of the society we live in. With this form of belonging, we allow ourselves to be one step removed, to take the time to be in solitude, to not take the messages and stories we tell of our work and schools and workplaces as self-evident truths, but to look at them for what they are, and to take the action, using Audre Lourde’s words, that ‘seeks a now that can breed Futures’, where we all belong.
So perhaps this is a second answer to my opening question. By organising in machine learning, and by queering machine learning, we build collective community and collective strength that makes it possible for belonging and loneliness and solitude to co-exist and strengthen each other for the benefit of our field.
As a final answer, I think I’ll instead recognise something more simple: that there is joy in being together. Joy in the possibilities of technology and what it can do in the world is what brought us to machine learning, and joy is what we see, whether in person or virtually, when we meet each other at this Queer in AI and all the others that have and will come. Joy can create a world of diversity and difference that we can be proud of, and proudly defend.
I posed that opening question: why do we organise, for two reasons. Firstly, to see that the queering of machine learning gives a unique view on technical systems and their design and deployment, that is our strength. And secondly, to join with you to create a community of nurture and belonging, where safety and loneliness are not to be feared, but used to create a more critical and responsible field of machine learning.
The global coronavirus pandemic, our resistance to racial injustice, and our concern for queer communities everywhere reminds us that to insist on belonging, community, and human connection, is as important today, and in our field, as it has ever been. Because there is joy in our belonging.
I’ll end here by reading the last few lines of a poem by Walt Whitman, We two, how long we were fooled.
...
We are what the atmosphere is, transparent, receptive, pervious, impervious,
We are snow, rain, cold, darkness, we are each product and influence of the globe,
We have circled and circled till we have arrived home again, we two,
We have voided all but freedom and all but our own joy.
Thank you.

To read more on this theme, read a short piece on Queer Exceptionalism in Science, and on race in Racialised Lives and the Life Beyond, or thoughts on A New Consciousness of Inclusion in Machine Learning.