Was grateful to be given the opportunity to support the 2020 TechAide AI4Good conference. This is the text of the talk.
You can find the video here.
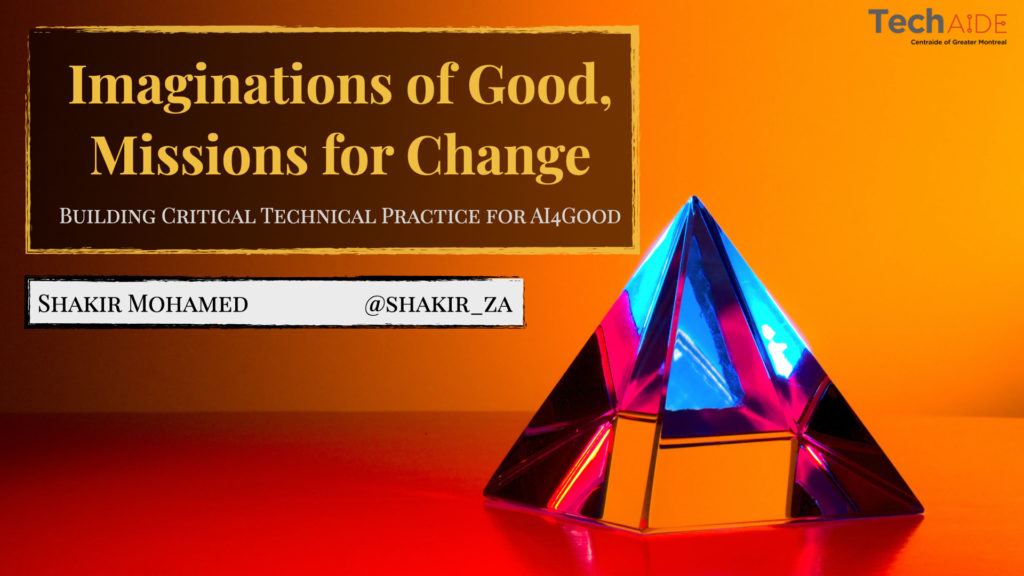
Greetings! It’s so great to be with you here today as part of the 2020 TechAide AI4GOOD Conference. My name is Shakir Mohamed, and my pronouns are he/they. I've entitled this talk Imaginations of Good - Missions for Change, and is meant to be both a conversation and a provocation: to engage with you in considering how we might build a new type of critical and technical practice of AI for Good, which is of course the theme of this year’s conference. The conference has a fulfilling mission and I’m grateful to the organisers for giving me the chance to support their charitable aims in this way, and hope you will be able to support the cause in any ways you can.
A little bit about me as we begin. I am a researcher at DeepMind in London, and a founder and trustee of the Deep Learning Indaba. You’ll find me supporting efforts for transformation and diversity. Some da ys I’ll be writing on methods for statistical inference and on others about socio-technical factors in AI. I am lucky to be able to do this, especially since I find so much joy in being able to work across disciplinary fields and with so many different methods of research. I’m interested in the types of mixed and messy questions and conversations that arise from this interdisciplinary approach to research, because I believe it is one way that we can be intentional and clearer about the good we want to see in the world, particularly in relation to AI and digital technologies. Rather than exploring some of my technical work and applications, I will instead rely on my work and experience that speaks to the questions of AI4Good. I’m so grateful for your time, and thank you for taking this journey for the next 25mins or so with me.
“Mankind barely noticed when the concept of massively organised information quietly emerged to become a means of social control”. I’d like you to guess when this might have been written. Given the increasingly concerning news items and so much incredible recent scholarship, you might guess that this is a recent description of algorithmic harms that emerged over the past two decades. You would be wrong. This is Edwin Black’s description of the world of 1900, and the invention of the punch card and a new wave of automation and data collection.
During this period, the little-known fields of computing and statistics, the pillars of today’s field of AI, became turbo-charged with the ability to sort through large amounts of data. With enhanced statistical ability, we were now able to accurately, and at the scale of nations and continents, identify objects of interest, assess tradeoffs between competing choices, organise the best sequence of actions, and finally audit the efficiency of any chosen course of actions.
This was a boon for almost every sector. The possibilities of good and imaginations of new types of futures that were now possible must have been exhilarating. Not unlike what we feel as AI technologists and scientists today. But, what if this enhanced statistical ability and new actions and efficiencies were used for the murder of Jewish people.
This is how statistics, and the tech industry of that age, entered its dark phase and turned towards evil ends and genocide. As Edwin Black forensically documents, for Nazi officials and throughout the second world war, statistics became “invaluable for the Reich”, and the Reich hoped to “give statistics new tasks in peace and war”. By abstracting away from the context of its work, computational science uncritically advanced its methods for greater accuracy, efficiency and financial profit—and enabled the death of millions. History reminds us that technological evolution tends to set ablaze high hopes coupled with cruel capabilities. Do we remember this foundation of our field of AI?
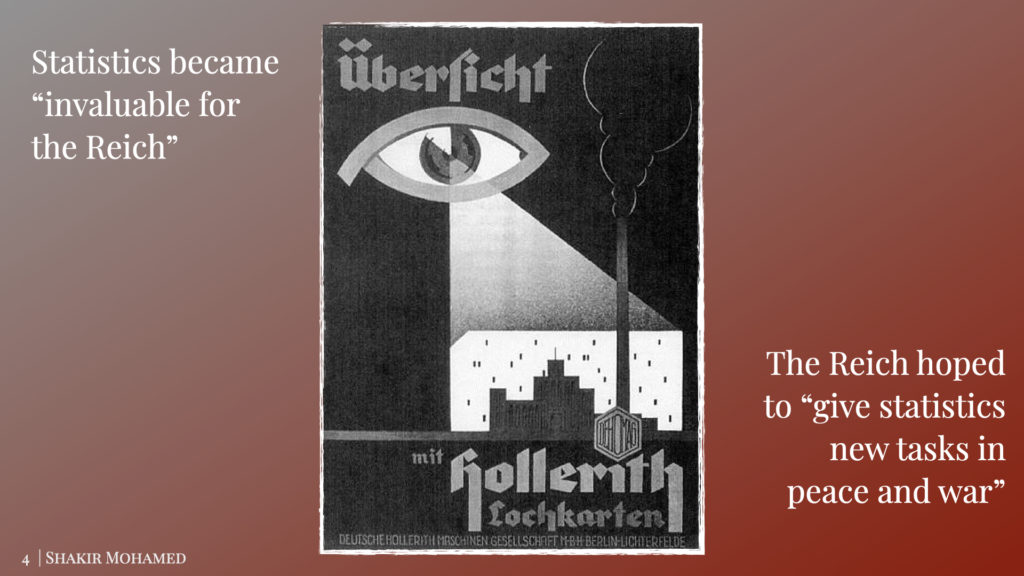
I’m telling this story to open up a discussion about the many concerns enveloping the project of AI4Good that have been raised in recent years. The concerns and objections raised about AI4Good are many. These include:
- The pet-project critique. That projects under the banner of AI4Good are not more than researcher’s pet projects in applied domains without any real intention for impact.
- The hype critique. That AI4Good advances a techno-optimistic and techno-solutionist view of the world that promises prosperity for society, but with very little evidence that this has ever been the case.
- The exploitation critique. That the project of AI for good is a veneer we put over technical products as part of its marketing strategy. Here, The project of AI for good is not aimed to meaningfully learn and engage with the communities it serves, but cloaks the real aim of data collection and profit.
- The vagueness critique. That our approach to AI4Good is enacted without specificity, and lacks a rigorous foundation in politics or social change, and in this way, consistently fails to engage with what it means to be ‘good’.
All these critiques have a strong basis of evidence for us to take seriously. Like the story of statistics and the Reich, these critiques shake and destabilise our confidence as technical designers - and open us to the possibilities that our work can instead be implicated in harm. This destabilising of our own confidence as scientists and technologists, for me, must be part of the approach we take in creating that cousin of AI4Good, responsible AI. And responsibility must be what we are all thinking and hope to mean when we use the word ‘good’.
It would be wrong to be all doom and gloom about the project of AI4Good. We do find many examples of great work that inspires us deeply into what is possible with contemporary AI. And when we find these instances, they point us to the paths of alterity that are already possible. I’d like to draw on three examples to dig a bit deeper into this question of good with you.
The first is the challenge of documenting and providing an evidentiary basis relating to human rights abuses. Amnesty International has shown that remote sensing data combined with artificial intelligence can be a powerful way to scale up human rights research. In their work they are able to shed light on overlooked abuses, and by sorting and classifying large volumes of images, are able to document, for example, the rate and the extent of village destruction and abuses. In this way we see technical tools developed in all the ways we hoped, supporting specific areas of need.
Worldwide, 9 out of 10 people breathe unclean air. Unfortunately, this is mostly happening in low-income countries. This is where the amazing work of AirQo comes in to provide accurate predictions of air-quality using a custom-built network of low-cost sensors that collects data across 65 locations in Uganda. With the COVID pandemic, non-pharmaceutical interventions are a concept we have all become acutely aware of, and air quality predictions provide another route for such interventions. The power of this incredible work lies in its potential to help citizens and governments better plan and mitigate changing environmental conditions, and these are the types of policy support we will continue to need more and more of.
The Serengeti-Masai Mara ecosystem is one of our world’s last pristine ecosystems, and the place that supports one of our world’s great animal migrations. This project by Snapshot Serengeti uses images from camera-traps to help conservationists learn which management strategies work best to protect the species that call this area home. Machine learning has helped to accelerate the study and analysis of the images collected, helping develop new theories of ecological function and a better understanding of the secret lives of the animals of the Serengeti. I was lucky to visit this region in 2019, and I thought i’d share this video of my own experience of this great spectacle of nature.
During the discussion, please share your own examples of projects that shine the light on what is possible and good. One common feature of the three examples I chose here is that they are centred on a clear mission for change. The mission to strengthen evidence for human rights, the mission to support public health with better environmental understanding, the mission to safeguard the world’s pristine ecosystems. These examples are not about projects seeking to do AI4Good. For them, AI4Good is a consequence of working towards a clear mission for change. And this is my first point of conversation for you: that we can address the critiques of AI4Good by putting in place the strategies that destabilise our confidence as technical designers by first, and always, centring the mission for change we are supporting.
For anyone working in the charitable and non-governmental sector, this is eminently familiar, and from their experience and methodology we can learn a great deal. I was lucky to be part of a collective to explore and develop these ways of thinking that we wrote about in a short paper. The key tool used in these areas is to develop and communicate a Theory of Change. The theory of change is often communicated as a diagram that maps the current state of the sector we are working in to the desired change we hope to see in the world. While the Theory of change is a requirement in the charitable sector, I think it can take a powerful role as a tool for shaping research and AI4Good.
By using a theory of change we can directly address the critiques I reviewed earlier. By making explicit the mission for change, we ensure the discussion about the political and social basis that forms the definition of good in our work takes place at the beginning of the work, and then throughout the lifetime of any project. The definition of good then becomes tied to the change mission. As we all know, for any piece of work, there are also competing factors and methodologies, and by plotting the path from a current state to a desired vision, these alternatives are also debated and discussed. It is also important that the Theory of change recognises that we don’t work alone and that we are not the only actors in any area we work in. So humility of approach is important, and any piece of work must assess how any ultimate change can be attributed to the work or an intervention.
This last point is especially important since it emphasises the role of measurement in assessing whether or not good outcomes have been reached, and being explicit about beneficiaries and who is ignored: assessing for whom the outcomes are good. A theory of change aims to be a causal graph from programmes and interventions to measures and outcomes. When charting this course of change, measurement can be thought of at every place in the causal chain. Although, Measurement is hard.
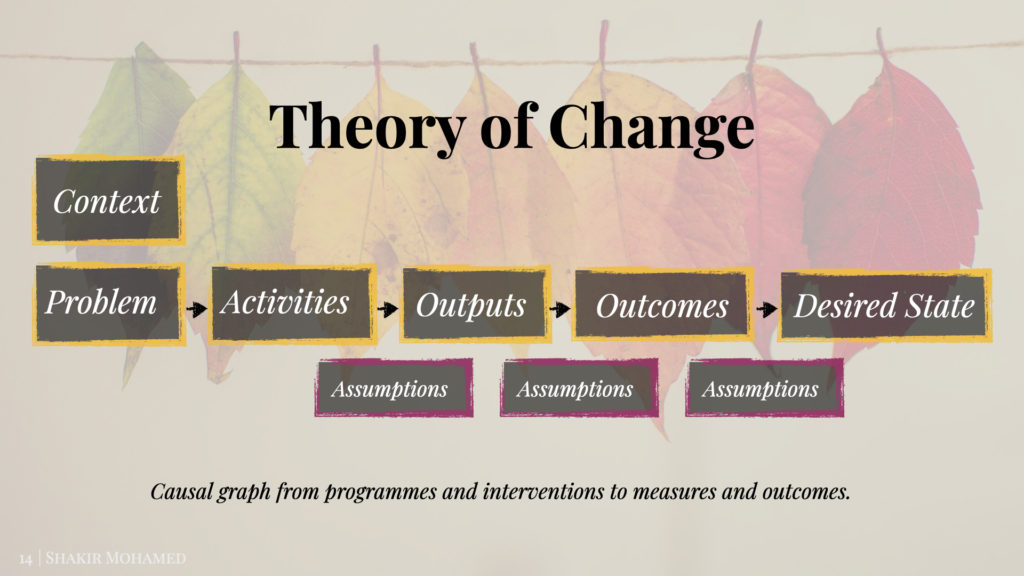
The sustainable development goals represent for us today one important source of guidance on AI for social good. One of the advantages of the sustainable development goals is that they come equipped with a set of 210 measures across the 17 goals. At the same time this is one source of criticisms of the SDGs, questioning the usefulness and reliability and frequency of these measures to enable reporting and change. In this general area, we are lucky to be able to take advantage of the knowledge and expertise around monitoring and evaluation developed in the charitable sector. As an aside, I think the theory of change is a powerful tool for research planning in general, since it creates a collaborative opportunity to consider our research programmes holistically and the many different types of contributions and outcomes that can come from our research.
Now switching gears a bit. The question I’d like to pose to you now is a simple one: is global AI truly global? This is a suggestive question, because for the most part AI is not global. Instead, it is localised and contained in specific individuals, organisations, and countries. And perhaps we can say this of global Science as well. We can shine a light on this by looking at the number of researchers in different countries as one rough proxy. Here in the United Kingdom, or in similar countries like Germany or the United States we find around 4000 researchers per 1 million people. In South Africa, where I am proudly from, this number is 400 per million. And in Ghana, the number is closer to 40 per million.
It is easy to become tempted, as part of the grand vision of a theory of change, to create a universalising view of applicability and of the advantages of the technology we hope to eventually develop. But this simple review of researcher numbers shows that the contributions to global knowledge are at present far from uniform. So any view on universality of AI needs to be more deeply questioned, and it is starting with this realisation that we can make a deeper theoretical analysis of the project of AI4good.
Since many AI4Good projects target social, humanitarian or developmental needs, questions about how they imagine, understand and use knowledge become the core area of interrogation. Our epistemological bases and Implicitly held and unquestioned beliefs about knowledge reveal themselves in the attitudes taken when doing research and deployment.
Attitude 1 - Knowledge transfer. By their nature, AI4good projects implicitly or explicitly acknowledge that knowledge and expertise is imbalanced in the world. For AI4Good, part of our work seemingly becomes to assist the migration of knowledge from centres of power (like research laboratories) to places where it is lacking.
Attitude 2 - Benevolence. An implicit attitude that emerges is that, where information, knowledge, or technology is lacking, technical development should be undertaken by the knowledgeable or powerful on behalf of those others who are to be affected or changed by it.
Attitude 3 - Portability. Knowledge and representations applied to any particular place or situation are believed to be just as easily applicable to any other situation or place, and that knowledge schemes developed anywhere will work just as well anywhere else.
Attitude 4 - Quantification. An inevitable conversation is that quantification and statistical accounts of the world as a tool for comparison, evaluation, understanding, and prediction is the sole way of understanding the world.
Attitude 5 - The Standard of Excellence. As a last attitudinal concern, do we assume the standards and forms and the world within our research labs in our metropole - so that is, within our centers of knowledge and technical power - are to be models of the future for other regions.
It is not always easy for any of us to question where these attitudes come from, but it is something we must do. These attitudes are partly the remnants of an older way of living and thinking forced on us all by our shared experience of colonialism. Colonialism was amongst the last and largest missions undertaken under the banner of ‘good’ - ostensibly to bring civilisation and modernity and democracy and technology to those that did not have it. Colonialism’s impacts continues to influence us today:
- physically in the way our borders are shaped, psychologically in how we think about ourselves and each other,
- linguistically in the role of English today as the language of science and exchange,
- by racism and racialisation that was invented during the colonial era to establish hierarchical orders of division between people,
- economically in how labour is extracted in one place and profit generated elsewhere,
- and politically within the structures of governance, laws and international relations that still fall along colonialism’s fault lines.
We refer to colonialism’s remnants and impacts on knowledge and understanding in the present using the term coloniality.
So, my second point of conversation for you, is to contend with the Coloniality of AI4Good. Coloniality seeks to explain the continuation of patterns of power between coloniser and colonised—and the contemporary remnants of these relationships. Coloniality asks how power relations shape our understanding of culture, labour, intersubjectivity and knowledge. When projects for good fail, what is found are default attitudes of paternalism, technological solutionism and predatory inclusion.
The approach I used to unpack those the attitudinal concerns relating to knowledge, follows one particular route of thinking about the decolonisation of knowledge. In this view of decolonisation, we are asked to reappraise what is considered the foundation of an intellectual discipline by emphasising and recognising the legitimacy of knowledge that has been previously marginalised. This realisation will lead us to make what is often called the decolonial turn, and for our field of AI, I have phrased this as a question of how we reshape our work as a field of decolonial AI.
Despite colonial power, the historical record shows that colonialism was never only an act of imposition. There was usually also a reversal of roles, where the metropole had to confront knowledge in the colonies and take lessons from the periphery, and in all spheres of governance, rights, management, and policy. A reverse tutelage between the centre and periphery was created, although with a great deal of loss and violence. By turning this insight into a tool we can use, a modern critical practice seeks to use a decolonial imperative to develop a double vision: actively identifying centres and peripheries that makes reverse tutelage part of its foundations, while also seeking to undo harmful power binaries: of the powerful and oppressed, of metropole and periphery, or scientist and humanist, of natural and artificial.
Reverse tutelage directly speaks to the philosophical questions of what constitutes knowledge. There remains a tension between a view of knowledge as absolute and of data that, once enough is collected, allows us to form complete and encompassing abstractions of the world, versus a view of knowledge that is always incomplete and subject to selections and interpretation under differing value systems. Deciding what counts as valid knowledge, what is included within a dataset and what is ignored and unquestioned is a form of power held by us as AI researchers that cannot be left unacknowledged. It is in confronting this condition that decolonial science, and particularly the tactic of reverse tutelage, makes its mark.
Reverse pedagogies create a decolonial shift from paternalism towards solidarity. This gives us two immediate tactics. The first is to create systems of meaningful intercultural dialogue and new modes of broader participation in technology development and research. Intercultural dialogue is core to the field of intercultural digital ethics, and asks questions of how technology can support society and culture, rather than becoming an instrument of cultural oppression and colonialism. Rather than seeking a universal ethics in our work, such dialogues will lead to an alternative in pluralism, and what are referred to as pluriversal ethics.
A second tactic lies in how we support new types of political community that are able to reform systems of hierarchy, knowledge, technology and culture at play in modern life. As one approach, I am a passionate advocate for the support of grassroots organisations and in their ability to create new forms of understanding, elevate intercultural dialogue and demonstrate the forms of solidarity and alternative community that are already possible.
I would like to share my own experience of putting this theory of community into practice. Around 4 years ago, I was part of a collective of people to create a new organisation, called the Deep Learning Indaba, whose mission is to strengthen machine learning across our African continent. Over the years of our work, we have been able to build new communities, create leadership, and recognise excellence in the development and use of artificial intelligence across Africa. And what a privilege it has been to see young people across Africa develop their ideas, present them for the first time, to receive recognition for their work, and to know amongst their peers that their questions and approaches are important and part of the way they are uniquely shaping our continent’s future. And I am proud to see other groups having followed in the same vein, in Eastern Europe, in South-east Asia, in South Asia, and South America - in addition to other inspirational community groups like Data for Black Lives, Data Science Africa, Black in AI and Queer in AI: all taking responsibility for their communities and building grassroots movements to support AI, dialogue and transformation. Looking back over the last 5 years, I believe we can now honestly say that global AI is now more global because of the commitment and sacrifices of these groups.
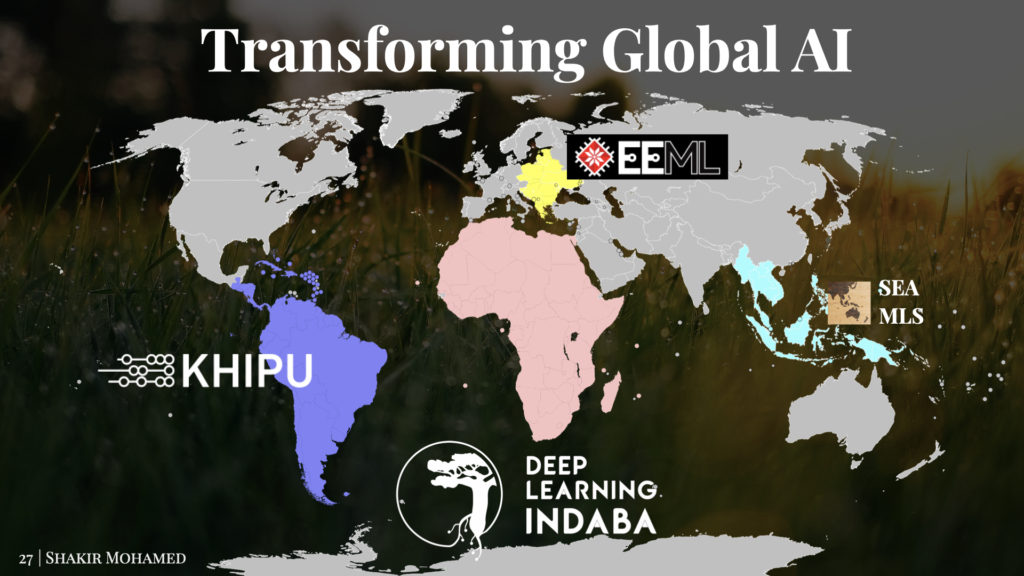
As I reach the end of our journey together, I’d like to share one problem area with a clear mission for change, which forms this year’s Indaba Grand Challenge. The change mission I’d like you to consider is the eradication of neglected tropical diseases (NTDs). Approximately 1.4 billion people—one-sixth of the world's population—suffer from one or more NTDs, and they are neglected because they generally afflict the world's poor and historically have not received as much attention as other diseases. And this word neglect is important, since as a very common phrase highlights, “neglected diseases are also diseases of neglected people”. The role of coloniality is important to connect with here, since we must ask questions of why historically and in the present such neglect exists, not just for NTDs, but in so many areas where AI4Good is called on.
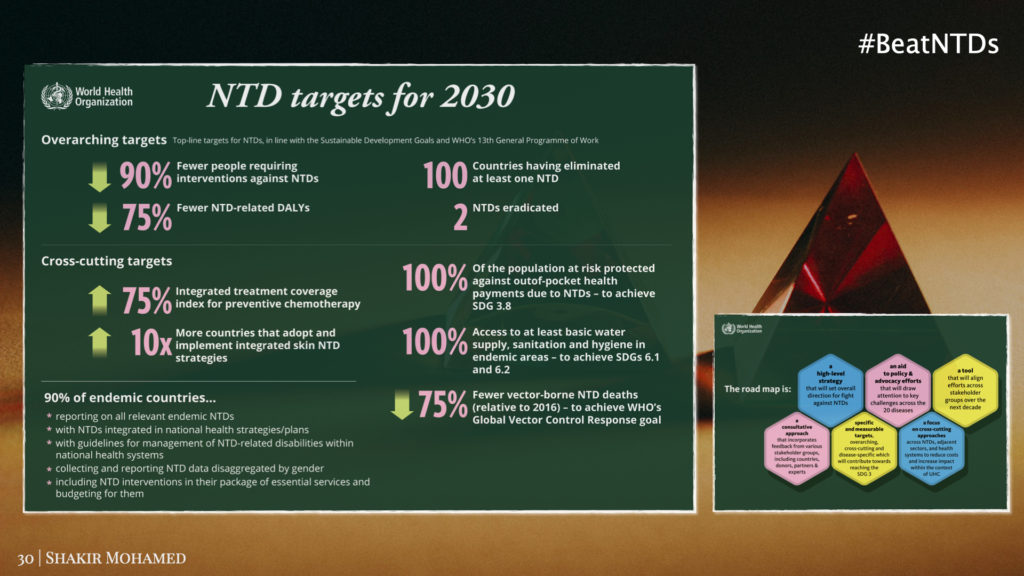
NTDs are a broad category of diseases, and I'm showing here the categorisation used by the world health organisation. We are lucky that 2020 has been an important year for neglected diseases, since the WHO has released an important new roadmap that builds dialogue, connects expertise and communities and clarifies the measurement needed to address this problem. As the WHO Director for neglected diseases, Dr Mwelecele Malecela said to me a few months ago, there is now an opportunity for machine learning and AI to help support this important mission, whether that is in the area of drug repurposing, or in diagnosis and detection, or physician training. There is so much to do in this space, and by creating a task for a specific disease for Leishmaniasis, the Deep Learning Indaba has tried to build a board coalition to help raise awareness and encourage research in this area: partnering with Zindi, Africa’s own data science competition platform, with InstaDeep, one of Africa’s leading AI and technology startups, with DNDi, the drugs for neglected diseases initiative, and the WHO, amongst many others to take a first step in this direction. My pitch to you is to consider the contributions you might be able to make with your expertise and attention towards any part of this problem.
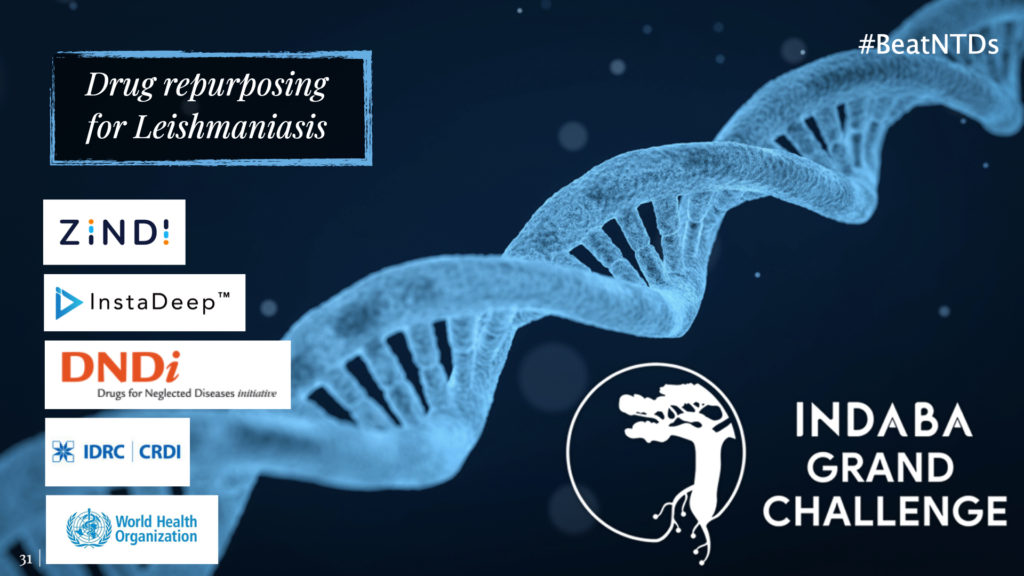
If I have accomplished my aims, then we have together reached this point, provoked by two streams of thinking:
- Firstly, That we must take seriously the criticism of the project of AI4Good, and can perhaps rescue it by recentring our AI4good efforts, instead of for a generic attainment of good, but towards the attainment of a specific change mission.
- And secondly that to continue to be ahistorical in our approach to machine learning risks reproducing harms of the past. How we remember our past, and what we remember, determines how we see the future. We have the advantage of historical hindsight, and by taking a reflexive approach to AI that uncovers coloniality’s role in our work and systems, we can develop new tools and tactics of technological foresight and action.
The challenges we are experiencing across the world today during the covid pandemic has led us all to ask many questions of ourselves and our work. With these challenges has come a renewed sense of the importance of connections, of community, and of our collective prosperity. This year’s TechAide conference speaks to that important need. And I think we can still optimistically say, with reflexive and decolonial tools aiding us, that we can together use AI to make our contributions to the vision of a world without neglect, filled with prosperity, and filled with joy.
To end, I thought we could read a poem together. It’s one I use to capture the vision for my own approach to research and AI. It’s one of my favourites, and maybe soon one of yours. It's entitled: Machines by Michael Donaghy.
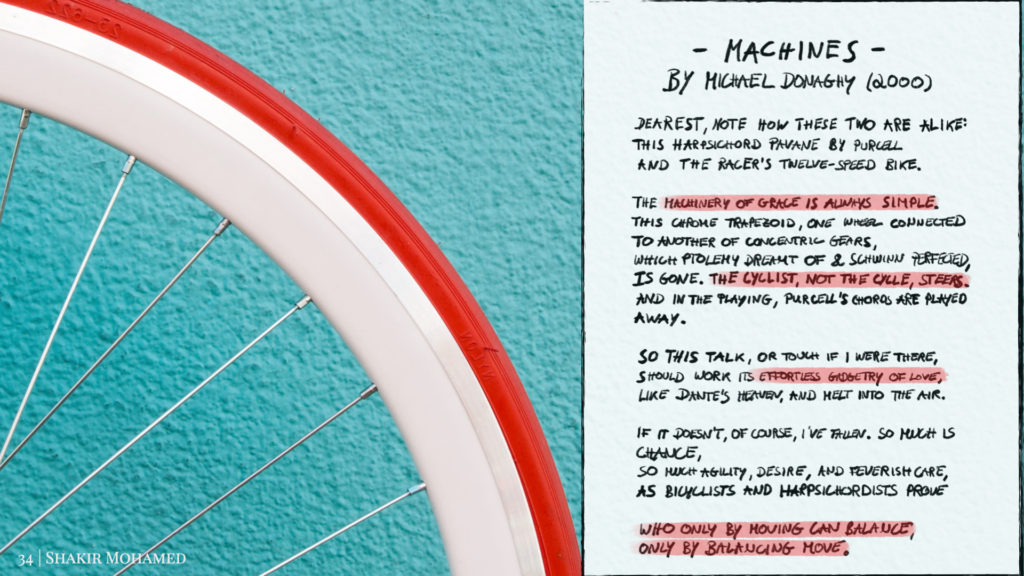
As a short postscript - some resources that might be of interest.
- Edwin Black’s forensically detailed book IBM and the Holocaust: The Strategic Alliance between Nazi Germany and America's Most Powerful Corporation.
- Kentaro Toyama’s Book: GEEK HERESY: Rescuing Social Change from the Cult of Technology
- Priyamvada Gopal’s incredible book of literary criticism: Insurgent Empire: Anticolonial Resistance and British Dissent
- And The Costs of Connection: How Data Is Colonizing Human Life and Appropriating It for Capitalism.
- And three pieces of writing I’ve contributed to:
This talk is part of a series that aims to collect money for Centraide, a non-profit dedicated to fight poverty and social exclusion. I urge you to click the link in the video to donate, and support them in fighting poverty and support social inclusion.