A Talk for the 2021 ICML Workshop on Tackling Climate Change with AI. I am extremely grateful to the organisers for the invitation. This is the text of the talk and also has links to the video.
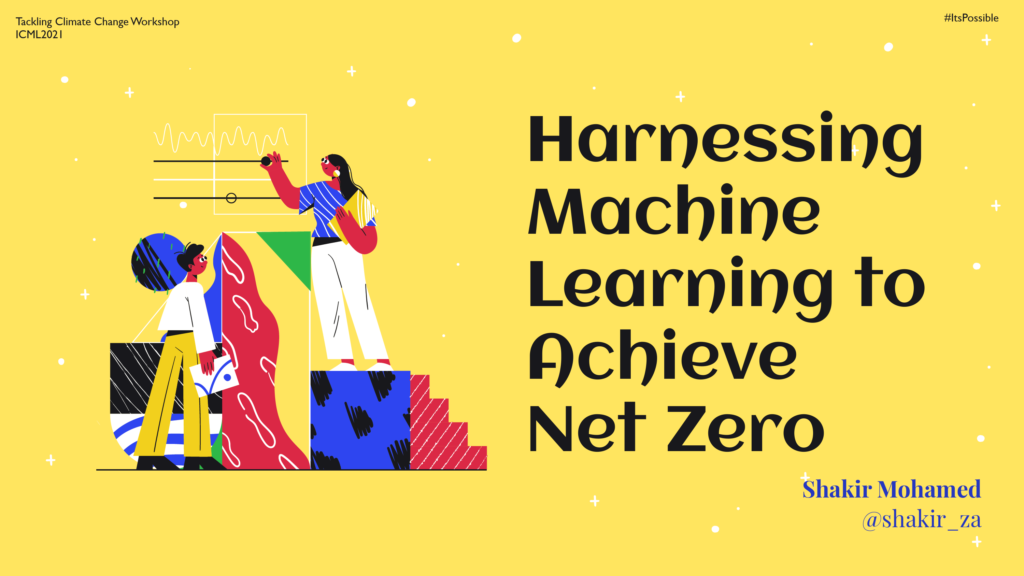
Hello everyone and thank you for being here today. This has become one of those must attend workshops over the years and I’m so excited to be part of the workshop today. To briefly introduce myself, my name is Shakir Mohamed and I use the pronouns he/they. I am a scientist and an engineer and I focus my efforts on machine learning to address global challenges, and also on developing the resources and thinking we need and that takes seriously the social and technical nature of our work. I’m extremely grateful to all the workshop organisers for thinking of me and for this invitation. It is committed action like theirs and of all the contributors to this workshop that is so essential to our future prosperity.
Our climate crisis continues, calling us all to consider what we hope, pray, expect our contributions to its resolution to be. We of course want to make contributions using the skills and in the scope of influence available to us, and so I thought we could hold a discussion on Harnessing Machine Learning to Achieve Net Zero.
At the outset, I do want to recognise that the climate change challenges we face can be addressed almost entirely using the technologies available to us today. So in that sense machine learning has little role to play. And while that might be the case, we do have a vital role in accelerating the many approaches for adaptation and mitigation that are needed, to support different forms of environmental monitoring and accountability, and to contribute to the evolution of climate solutions, especially for the different forms and formats they will need in order to be used in countries and societies across our planet. Environmental and climate science is also a rich source of problems, collaborations and data and as a research area raises fundamental questions that are essential in advancing machine learning research. As many commentators point out, if machine learning systems actually work on problems in the physical world and for our climate, then that is a special type of evidence that we are actually doing something right.
I’ll develop some ideas for your comment and discussion for the rest of the talk in 3 parts: Net Zero possibilities, machine learning in policy-making, and decolonial climate science. And hopefully all three of these themes will come together in some form when I conclude in 20mins or so.
Part I: Net Zero Possibilities
We are here at this ICML 2021, having witnessed Earth’s hottest decade in history. We are all used to seeing these stripe graphs that are increasingly red and reaching new highs. And the physical change of climate is clear to see. This graph is the instantiation of centuries of understanding of climate change, and is almost overwhelming when we think about the scale of response needed to meet the target of not exceeding the 1.5-2 degrees warming by the mid-century.
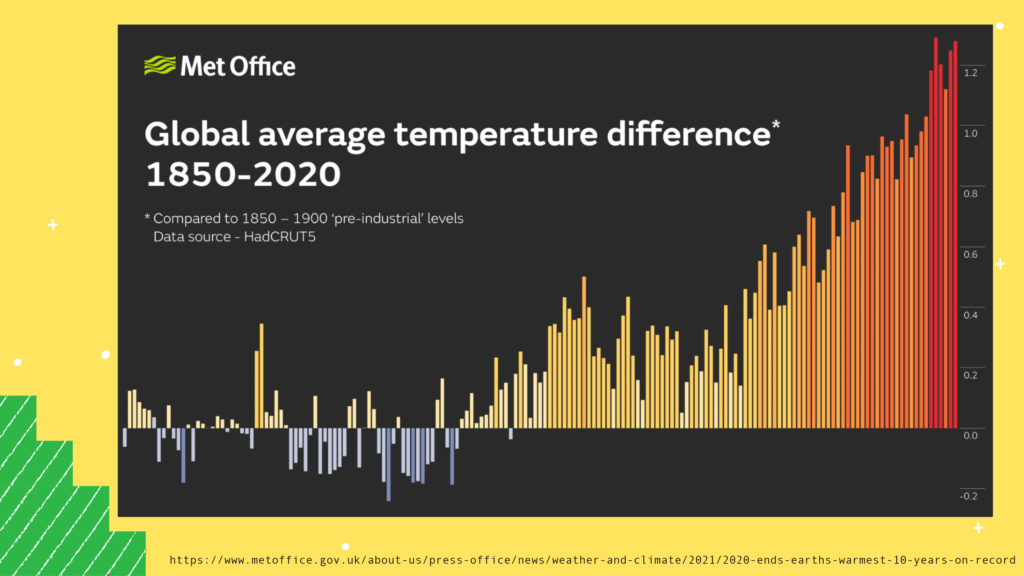
In another very recent observation. The city of Kyoto in Japan, having collected data starting from 800 CE, recorded in spring this year, the earliest peak of the cherry blossom flowering in 1200 years. Perhaps a seemingly benign change on this great spectacle of nature, but when we see the same change in the early flowering of fruits and crops while still at threat from winter frost and damage, this is far more serious, costing enormous amount of money and loss of livelihoods, threatens our entire food supply, and places at risk that most basic of humans needs.
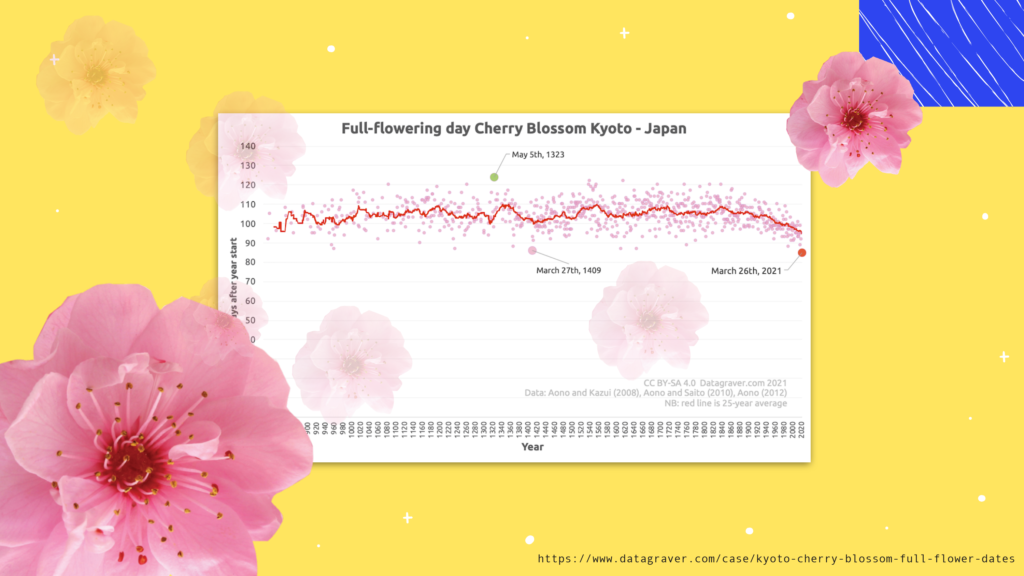
#DefeatDayZero. That was what the residents of Cape Town in South Africa rallied around as they faced their most severe drought in 2018 and the years preceding it. This created a monumental disruption, almost as if it were the plot of a disaster film. Day zero was the day that water reservoirs would become so low that the city would not be able to supply water to anyone, and was to be avoided at all costs. This mandated the most drastic behavioural change on the part of the city’s residents, businesses and visitors, with everyone needing to adapt to life on just 50L of water a day. Compare that to the average daily water consumption of 300l per day in the US, or 180l per day China, or 121l per day in Germany to imagine for yourself what that change implies.
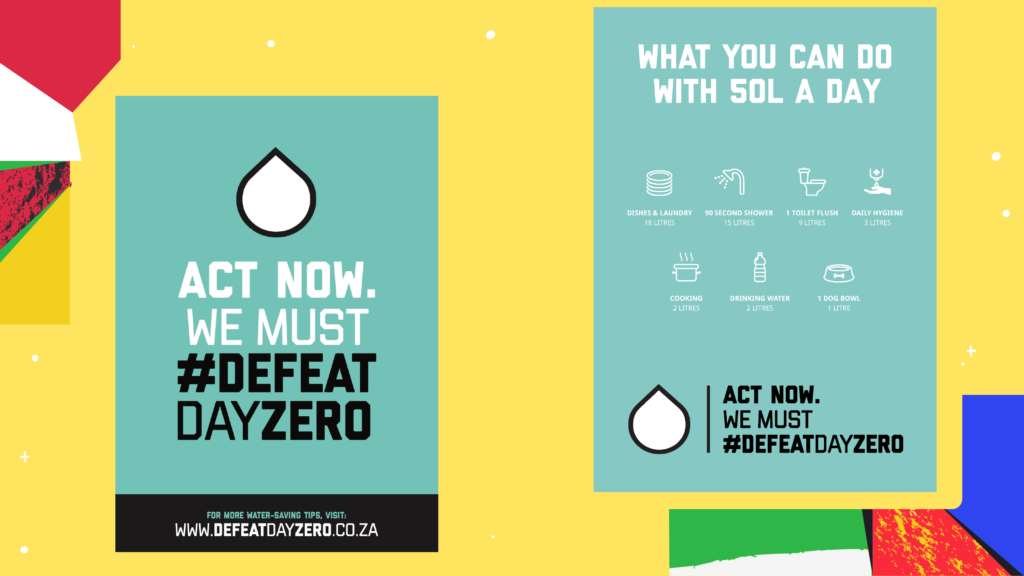
Staying with Germany, In February 2021, Germany’s forests were declared sick, with more trees having died in Germany in 2020 than in any other previous year. An overly dry-summer allowed the culprits, the bark beetle, to dig deeper into the spruce trees, but was part of larger environmental impacts from storms, drought and forest fires in previous years that together all affected the health of these great forests. And the consequence of this only starts with the 1.5B Euros that the german government will now be spending to support their restoration.
And i’m sure you can share so many other stories from your countries, telling the story of change to our landscapes and seas, to the lives of animals, and alarming change wherever we choose to look. Since we do face such changes, it is only natural that we would want to use the science at our disposal, and where we have expertise to help in the fight against climate change. A core solution to these problems is to reduce our carbon dioxide and other greenhouse gas emissions.
So that brings us to the concept of net zero. The concept of net zero is that we can balance the sources and sinks of CO₂ emissions, and in this way prevent further warming of the climate, allowing our planet to recover and restore. 131 countries have now set or are considering a target of reducing emissions to net zero by 2050. #ItsPossible. We are called to do everything we can, and in considerate and coordinated ways, to accelerate technologies and behaviours and adaptations towards net zero, and do all we can to remove the activities that slow or prevent its attainment.
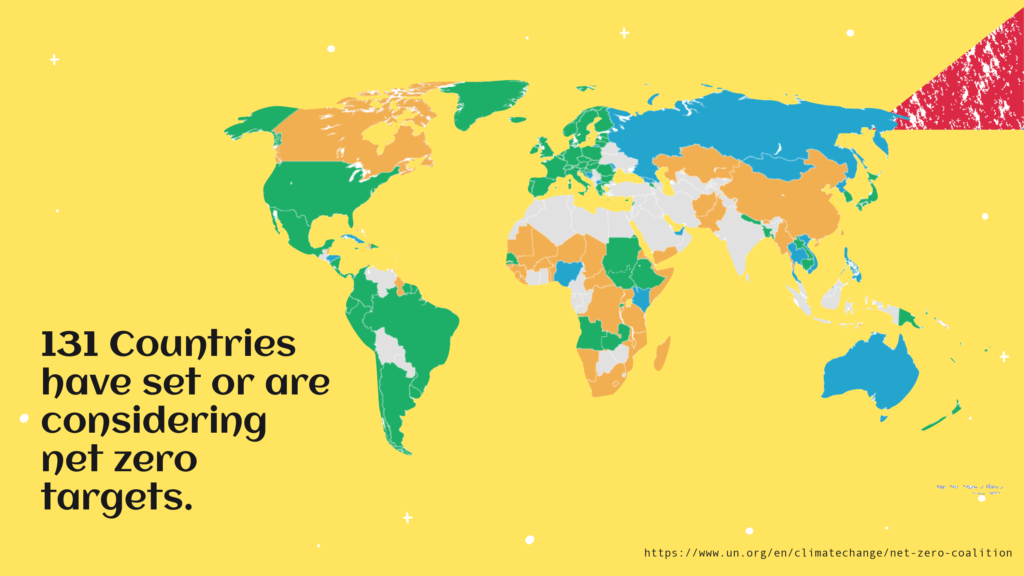
The Net Zero approach is not without criticism. There are concerns about language of “nature-based solutions” that allow for accounting towards net zero but without making any actual changes in emissions. Carbon that is put into forests creates competition for land, and there could be other side-effects of net-zero interventions. This is coupled with a critique of too much focus on carbon removal rather than on negative emissions, shifting our focus and investments in the wrong ways. We will need to think about these concerns as part of our broader sociotechnical concerns as we think about our contributions in this space. And I think data and reporting could be a helpful mechanism for accountability in such areas.
The solutions for net zero are many, and they make many opportunities for high-impact machine learning research. The biggest impact to net zero will come from decarbonisation by electrification. We’ve seen many examples of different areas:
from approaches for wind forecasting, that make predictions of wind up to 36 hours ahead. These predictions allow uncertainty in wind energy output to be used in the energy mix scheduling and provision on the grid.
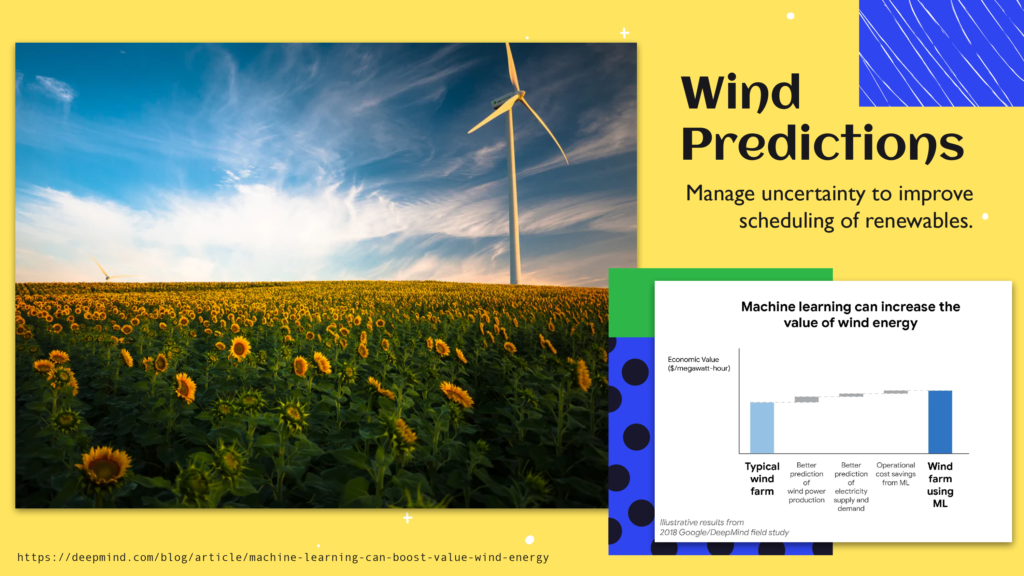
The focus on energy grids is vital, and will rely increasingly on tools for fault detection, demand forecasting and grid balancing, and there are some great papers on these topics at the workshop to look out for.
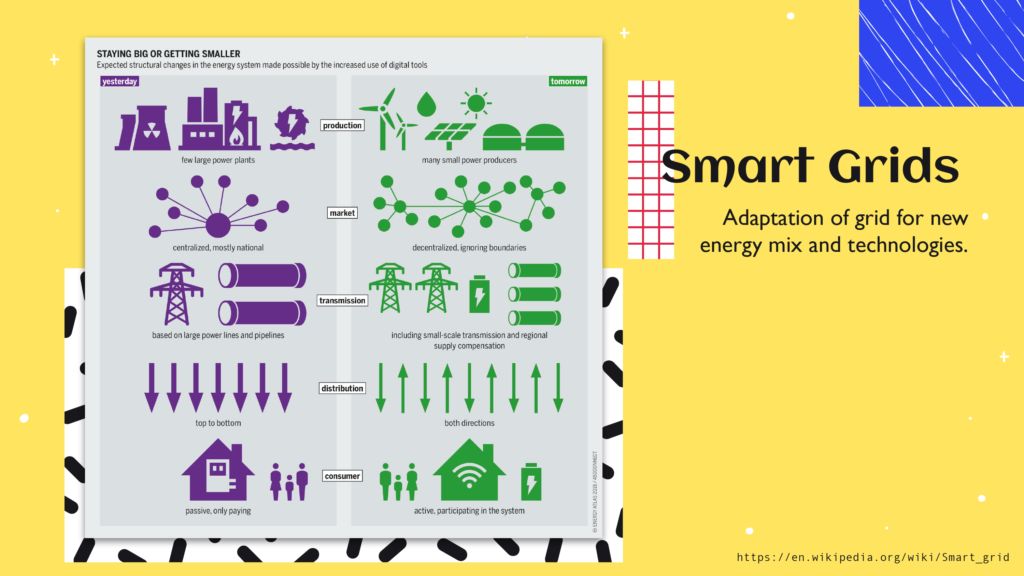
Hydrogen appears as one core part of an energy transition and decarbonisation approach. Hydrogen’s role is large: from energy supply and storage, its role in alternative fuels for heavy transport, shipping and aviation, and in the manufacture and use of different types of fertilisers. I haven’t seen projects on this, but this seems to me an important area for us to look into, and this relates to the broader applications of machine learning in industrial processes.
As a last example, the area of precision agriculture aims to provide whole-farm management that preserves resources and provides efficiency. There is a great deal of remote sensing data, whether satellite or multispectral observations, that are central for the decision-support tools that support these approaches.
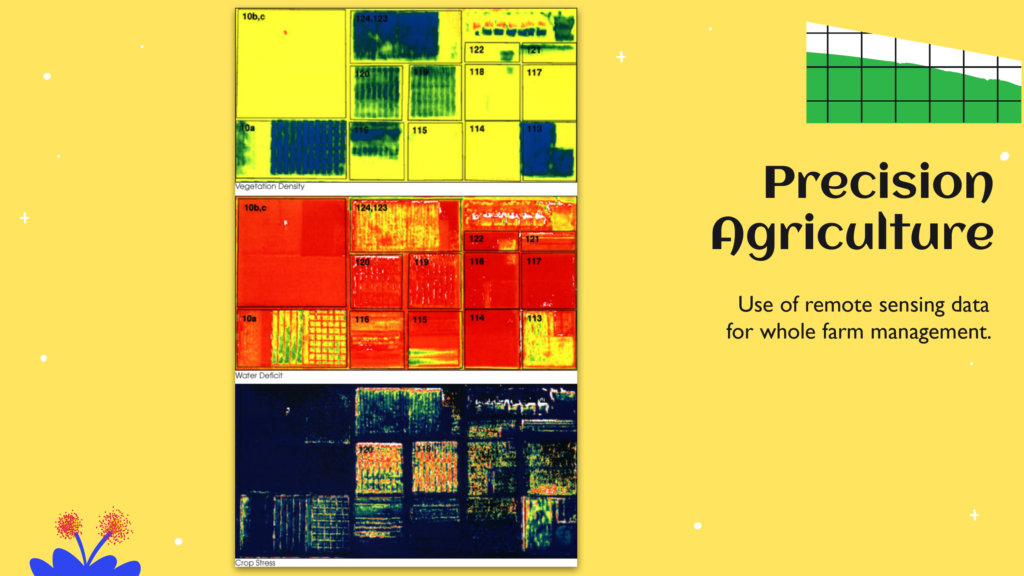
My own research in these areas has been a bit more removed from these direct applications, but more focussed on the general prediction of environmental variables that feed into these net zero solutions. Whether we are predicting rain, as in the problem of precipitation nowcasting, or other variables like wind or solar reflectivity, we do encounter challenging problems essential for advancing machine learning research. Data like radar contains events of varying frequency, and that is a fundamental and under-explored question: how do we develop rare event prediction models from high-resolution imaging? For weather phenomena that are fundamentally uncertain, how do we quantify and report those uncertainties? Deep Learning methods can be powerful, but we don’t yet have ways of incorporating physical constraints, meaning that their predictions can fail to satisfy the expectations we have of physical and temporal consistency of a prediction, making the need for new types of evaluation more important than ever. Environmental and physical predictions are important, since while we work towards net zero, weather-dependent decision-making, specifically for adaptation, will continue to be needed as all the examples of drought and hot decades and dying trees point to.
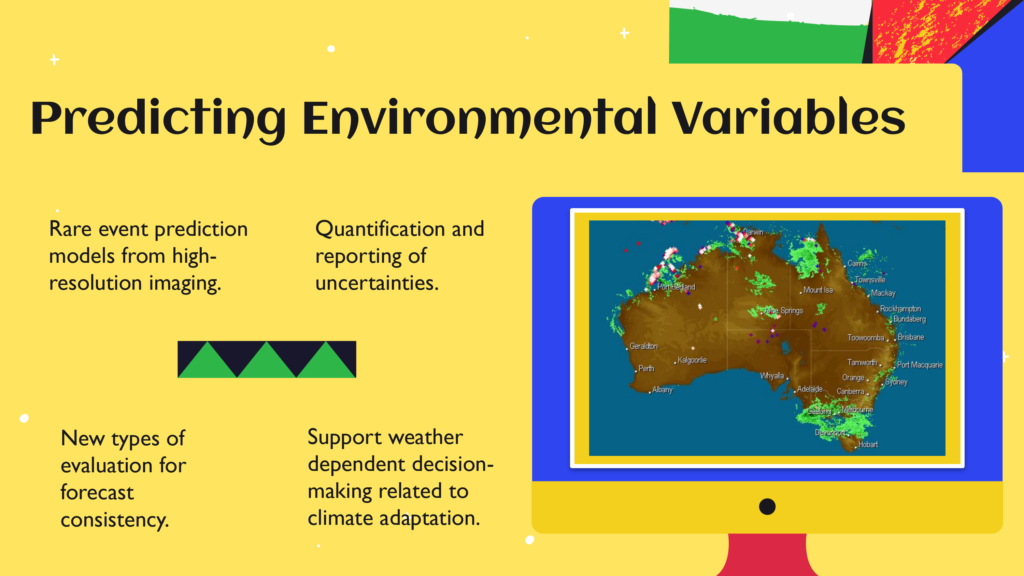
As a guide for us, let’s remember the words of one of our world’s greatest environmentalist, Wangari Maathai, who says to us:
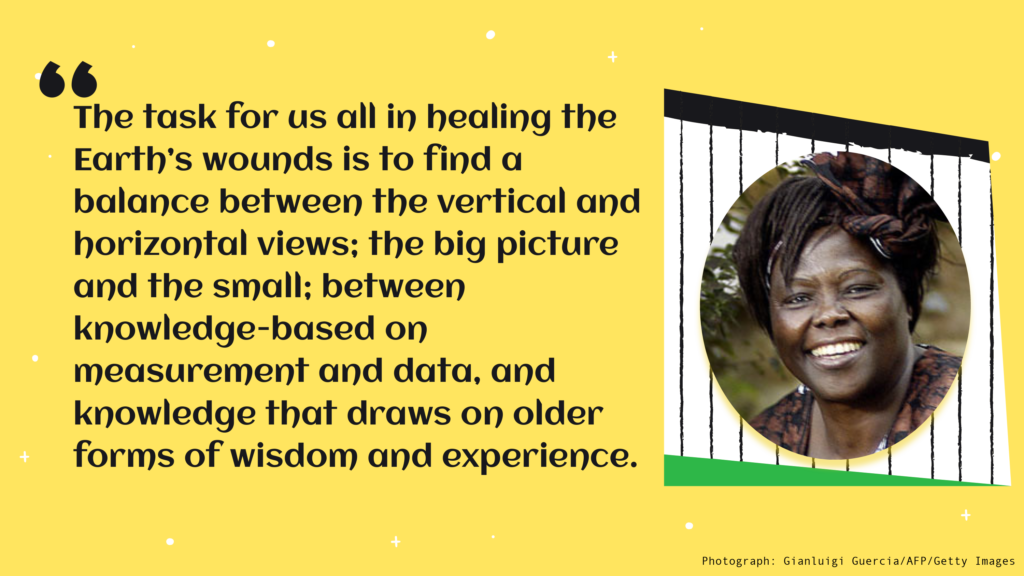
“The task for us all in healing the Earth’s wounds is to find a balance between the vertical and horizontal views; the big picture and the small; between knowledge-based on measurement and data, and knowledge that draws on older forms of wisdom and experience.”
Let’s build on Wangari Maathai’s call for exploring balance in our practice. So on to the next part.
Part II: Machine Learning in Policy Making
Beyond our technical contributions, I think there is an important role for us as scientists and engineers to be engaged in conversation with policy-makers and our governments. This is one part of a possible greater participation in the wider-ecosystem of machine learning. The word policy-making is very broad and can mean many things. It does mean an engagement with the politics of sustainability. Engaging with this politics is important because we clearly recognise the role of values in our technical work, especially the value of sustainability and living in harmony on our planet. And policy-making can mean many other things: working directly with governments, with trusted scientific academies, independent policy-focussed non-profits, or activism and direct-action networks. All of these are valid forms of engagement. And I think these engagements, beyond our technical roles, can be a great source of sustenance and joy for us all.
I want to share with you one piece of work in this vein that I was engaged in with the Royal Society on a policy project called Digital Technology for the Planet, which set out to clarify the role of digital technologies, which includes machine learning, and to put forward a set of policy recommendations, which focussed specifically on the UK government, but also included other more more wider recommendations.
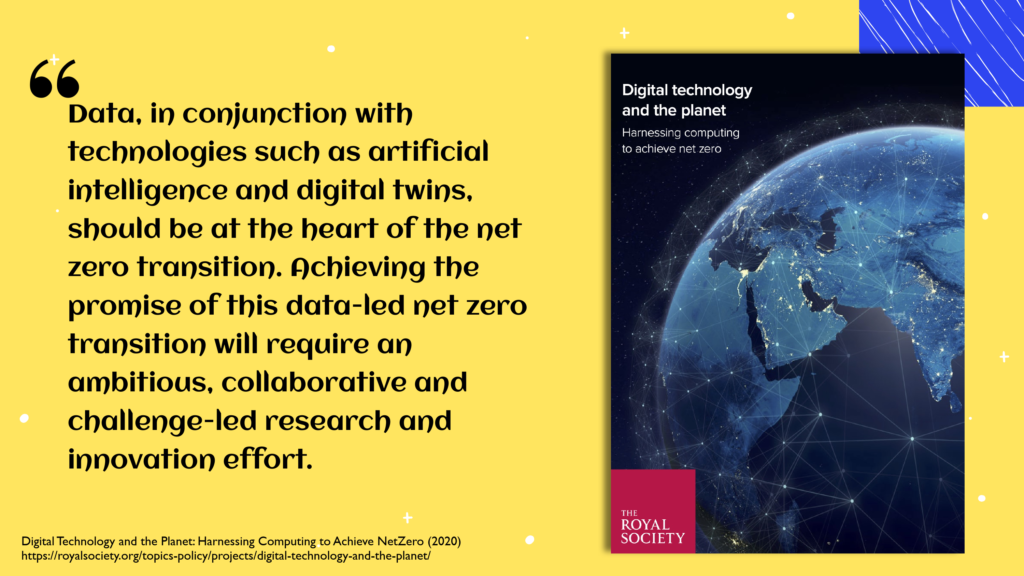
The Royal Society report on Digital Technology for the Planet develops the idea that ‘Computing for net zero’ could play an important role in global, regional and national net zero strategies. It aims to summarise the landscape and areas of policy intervention related to computing, data, security, trustworthiness, and research in this area. Like many policy recommendation efforts, it began with a series of evidence gathering exercises, in the form of a series of workshops with experts and researchers across several themes, including, achieving ‘net zero’ from buildings and land use, decarbonising supply chains and logistics, carbon emissions from digital systems themselves, and climate science and monitoring.
I encourage you to have a look at the report, which begins quite clearly with the idea that our transition to net-zero will be data-led. And in this effort, the report takes seriously the idea of digital twins and their role in achieving net zero. A digital twin in a simulation of physical objects, like wind turbines, railway systems, or energy grids. Anyone thinking about generative models and sim2real problems will be intuitively familiar with this idea. With widespread and reliable digital twins, we can imagine a control loop for the planet: that integrates data from systems, enables analysis and exploration of possible changes to the system, in safe, non-destructive and cost-effective ways, and then allows for corrective actions in the real-physical systems.
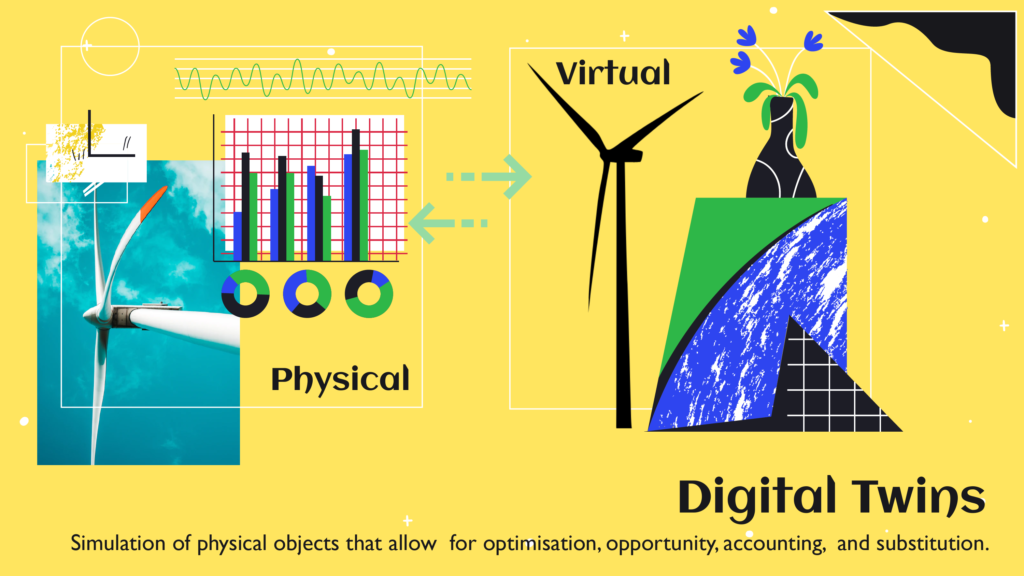
With a control loop for the planet, we can achieve different goals:.
Optimising, by using simulations to explore ways to reduce the carbon footprint of existing systems. Again consider the examples of wind turbines or precision agriculture.
Enabling: A control loop can make low-carbon systems possible, e.g., by the role of smart grids.
Accounting: that allows equitable distribution of the burden of emissions reductions, for example, by supply chain tracking and monitoring carbon trading. And finally
Substitution: by providing compelling alternatives to current technologies, e.g, through the role of intelligent transportation, or with electric or autonomous vehicles.
What is important about this control loop, is that none of this is about autonomous independent systems of environmental control, but sets of systems that are teamed with humans in intimate ways to meet the challenge of achieving net zero emissions.
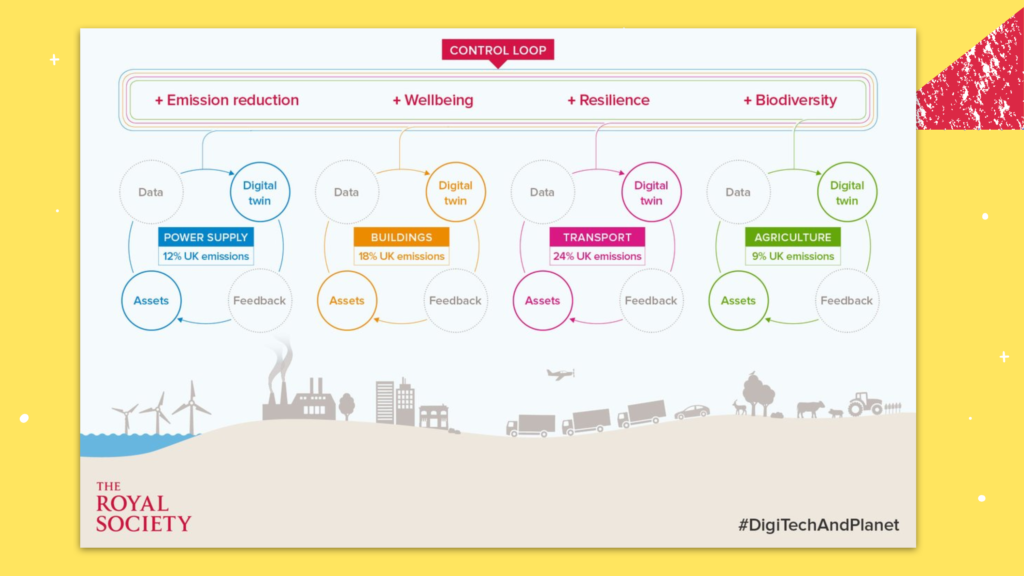
Part III: Decolonial Climate Science and AI
I think it is fundamental to give more deeper thought to the idea of “The planet” in the title of this report, and of questions of equity and redistribution, and the historical development of our climate crisis. We do need to keep asking why we are in this crisis, who’s imagination of the planet is being considered in these recommendations, who’s net-zero problems and research questions are we undertaking, and why. These are fundamental questions that emerge from taking a sociotechnical view of our field: a view that not only values technical advances, but deeply understands the role of society in shaping our work and that our work shapes society.
Formal colonialism may have ended, but its legacies remain with us today: in the way we think about ourselves and each other, in our countries names and borders, in the language of English we are using to communicate to each other in this workshop, and in the development of the climate crisis we face today. We refer to this legacy of the colonial mindset and structures and institutions using the word coloniality. And it is in considering the coloniality of machine learning and AI that we gain a powerful tool for considering and addressing these important questions, of why and for whom, I just posed to you.
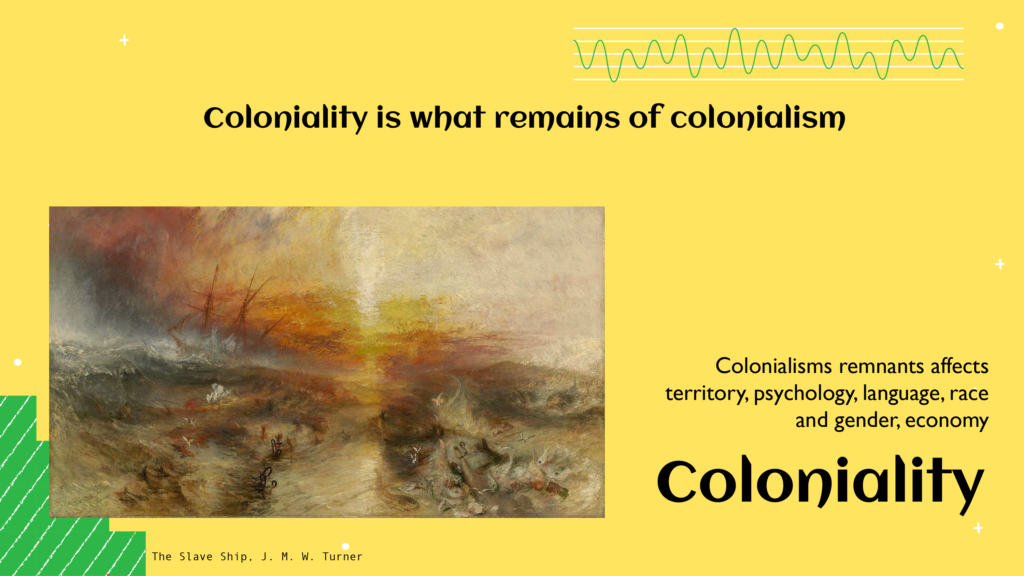
If you accept this position, and I hope you’ll engage with it further to reach your own conclusions, then we will be led to ask how we can turn towards a Decolonial AI. The harms that have been documented as a consequence of AI deployments across the world—whether in facial recognition, predictive policing, unequal resource distribution, shifts in labor practice—did not emerge by chance. They result from long-term, systematic mistreatment and inadequate legal and economic protections. Any pathway to prosperity people and the earth will have to contend with this legacy, and in particular with at least three distinct forms of algorithmic harm: algorithmic oppression, algorithmic exploitation, and algorithmic dispossession.
Algorithmic oppression describes the unjust privileging of one social group at the expense of others, maintained through automated, data-driven, and predictive systems.
Algorithmic exploitation construes people as automated machines, obscuring their rights, protections, and access to recourse—erasing the respect due to all people.
Algorithmic dispossession is the centralization of power, assets, and rights in the hands of a minority, through technological interventions or by institutions that shape regulatory policy.
All three forms of harms are one we must constantly assess in our applied work in climate and environment. Our drive for greater green energy through wind turbines has led to the interference of the reindeer migration and the dispossession of the indigenous Sami people in northern Norway. Wind farms necessitate the building of roads and other infrastructure, often in areas that were previously undisturbed by humans, and reindeer simply don’t go to places where there are turbines. As the Sami leaders so clearly say: The Sámi people are not the ones who have contributed the most to climate change, but we seem to be the ones who have to carry its greatest burden.
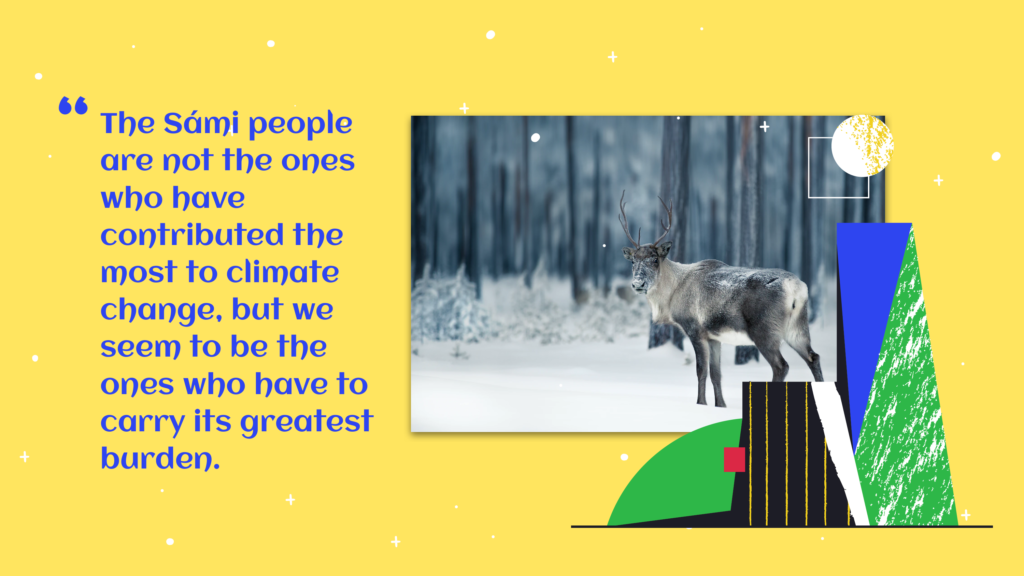
We also see other dispossession dynamics that exist in climate policy. Most policies and sustainability schemes have been largely shaped by the environmental agendas of the Global North—the primary beneficiary of centuries of climate-altering economic policies. These policies take very little account of ways of living or practicalities of people in Global south producing countries, nor are they involved in the development of such policies. So when we engage in these policy exercises, we need to consider our position and whose concerns and knowledge we choose to amplify or ignore.
One comfort many researchers take is that environmental data are not measurements derived from the social world, with the implication that many of the challenging social problems we’ve seen in other areas of machine learning, like algorithmic bias, are perhaps fortunately avoided. And while this is the case for many sources of data, we must continue to exercise caution. Energy poverty and the challenges of changing climate already fall disproportionately on the racialised and poor. Smart grid and the behaviours they regulates are social worlds, environmental data sets and benchmarks have an imprint of human choice by the people who create them, and the predictions we provide are about interactions with the social world and shape the behaviour of decision-makers.
As a last exemplar concern. Working towards net zero is not only a good thing for our climate, but also makes a very strong business case. As new net zero technologies are created, scaled and refined, they will become a large part of the economy of the future, creating new opportunities for exporting net-zero technologies to those that don’t have them, while creating jobs and wealth in the countries that do. This can unfold in many different ways, but perhaps the reluctant sharing of vaccines globally gives us reason to be concerned about how this technologically-enabled green economy of the near future will unfold.
In all these examples, we are asking who’s benefits are being protected. Whom are regulatory norms and standards designed to protect—and who is empowered to enforce them. What attitudes of benevolence and paternalism are at play in how we conduct our work. And what other, more participatory and inclusive approaches might we take instead.
Summary
I’m extremely grateful for the opportunity to have explored these seemingly disconnected themes with you and thank you all. There were three I wanted to bring together for your assessment and contestation:
Firstly, Net zero attainment is a crucial problem for machine learning researchers to direct our efforts towards. Not only are there important existential challenges to help solve, but the environmental sciences offer a powerful test bed for any work in machine learning, exposing fundamentally important questions about measurement, evaluation, rare event prediction, uncertainty quantification, and so much more.
Secondly, a sociotechnical approach to our work is vital, we must continue to see the technical and social world speaking to each other always. One way of enacting that role is through policy engagement and broad engagement, with many policy approaches supporting the role of digital twins, which is also a fertile area for research.
Finally, a failure to contend with historical hindsight risks us reproducing the harms of the past. We already see the coloniality of netzero unfolding, and our responsibility as scientists is to pose difficult questions and shape technological advancement in decolonial, inclusive and other ways, where the risks of new approaches are shared evenly along with the benefits.
As I do in so many of my talks these days, I’d like you to join me in reading a short poem that for me connects the many themes we have explored today. This is: The Question by Theo Dorgan:
When the great ships come back,
and come they will,
when they stand in the sky
all over the world,
candescent suns by day,
radiant cathedrals in the night,
how shall we answer the question:
What have you done
with what was given you,
what have you done with
the blue, beautiful world?
Some Resources that might be of interest.
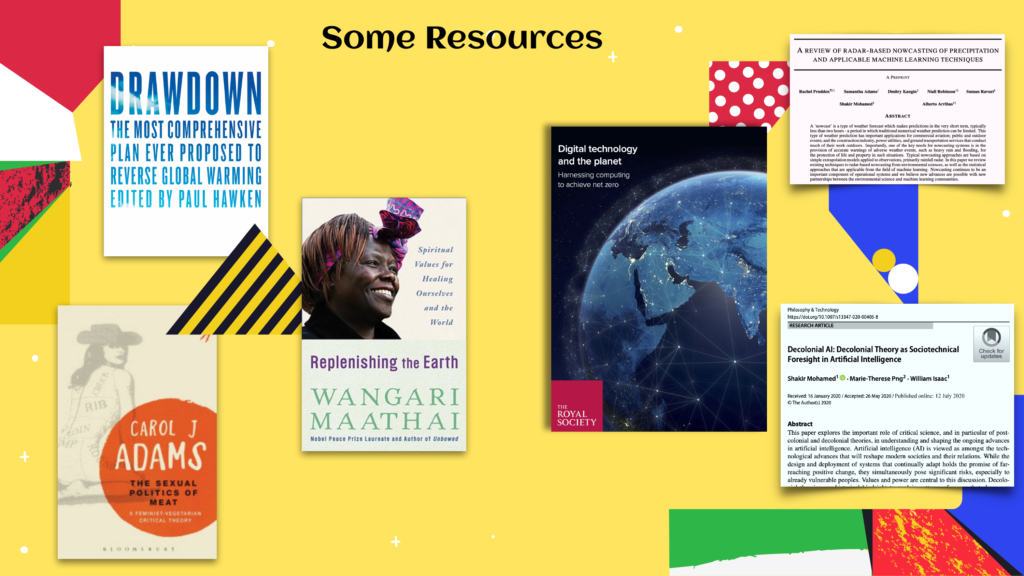